Brief
In evidenza
- Consumers often stray from their primary bank for new banking products, and this hidden defection erodes banks’ economics and customer relationships.
- Recent advances in analytics enable banks to activate, not just retain, their high-value customers.
- The analytics work best when informed by five principles: segmenting customers by value, automating forecasts, predicting loyalty, understanding what causes churn, and taking a test-and-learn approach.
Neobanks and other digital start-ups have been chipping away at banking markets for a decade. Their relatively transparent pricing and products make it easy for consumers to shop around. And with Covid-19 accelerating the use of digital channels for advice and sales, consumers are even more open to trying digital attackers.
In just a few years, for instance, Chime has built a bank in the US that has twice as many primary checking customers as Huntington, according to our NPS Prism℠ benchmarking data. Chime has attracted a mix of younger, low-balance customers and older, more affluent customers without a single branch or ATM of its own, or even a core banking technology platform.
Instead, Chime has built a sleek mobile app focused on what it calls Spending Accounts, with features such as early direct deposit and easy cashless payments. Opening a Chime account takes an average of 15 minutes—significantly faster than with competitors—and before customers even fund the account, they’re notified that a debit card is in the mail. In addition, Chime touts having no hidden fees, and it makes moving money simple. Chime’s model has paid off in customer advocacy: Its Net Promoter Score®—a measure of customers’ likelihood to recommend a store or brand—is among the highest in the industry at 66.
Defection is hidden
Incumbent banks might not even notice how neobanks and other firms are gaining share. Customer churn rates tend to understate the issue, as most defection in banking consists of people obtaining credit cards, loans, or other products elsewhere, but not closing their original account altogether. Maintaining a healthy base of primary customers may disguise the dire reality of declining share of wallet.
Retaining customers is not in itself a useful goal, being too little, too late. Indiscriminate retention hurts a bank’s economics, because it entails spending money on loss-making customers or focusing on customers not susceptible to intervention. One-off tactics often fail to produce lasting results and will be less effective than a systematic approach to loyalty that puts customer priorities at the heart of the business. Shifting customer needs and preferences account in part for customer leakage, so if the bank’s core offering fails to address these needs, its options are limited.
Recent advances in analytics enable banks and fintechs to activate their high-value customers more effectively, through granular segmentation and personalization. One finance company, for example, implemented a systematic activation program in pilot markets that yielded a 22% increase in customer retention along with a 4% lift in profitability. It is now rolling out that program across other markets.
Five principles for customer analytics
Banks that have started down this path are finding that their deployment of advanced analytics works best when backed by five principles.
Take stock of customers’ true value. Measures indiscriminately targeting large customer groups by age, deposit volume, or other variables tend to be counterproductive, inadvertently triggering churn or annoying disengaged customers or those whose spending is maxed out. Moving beyond crude segmentation, advanced analytics can help develop a more granular view of target customer groups that is anchored in their value. For example, a bank would break down its mortgage business based on the type of community and the complexity of the financing structure, or another combination of features determined to be statistically significant in affecting relative customer value (see Figure 1).
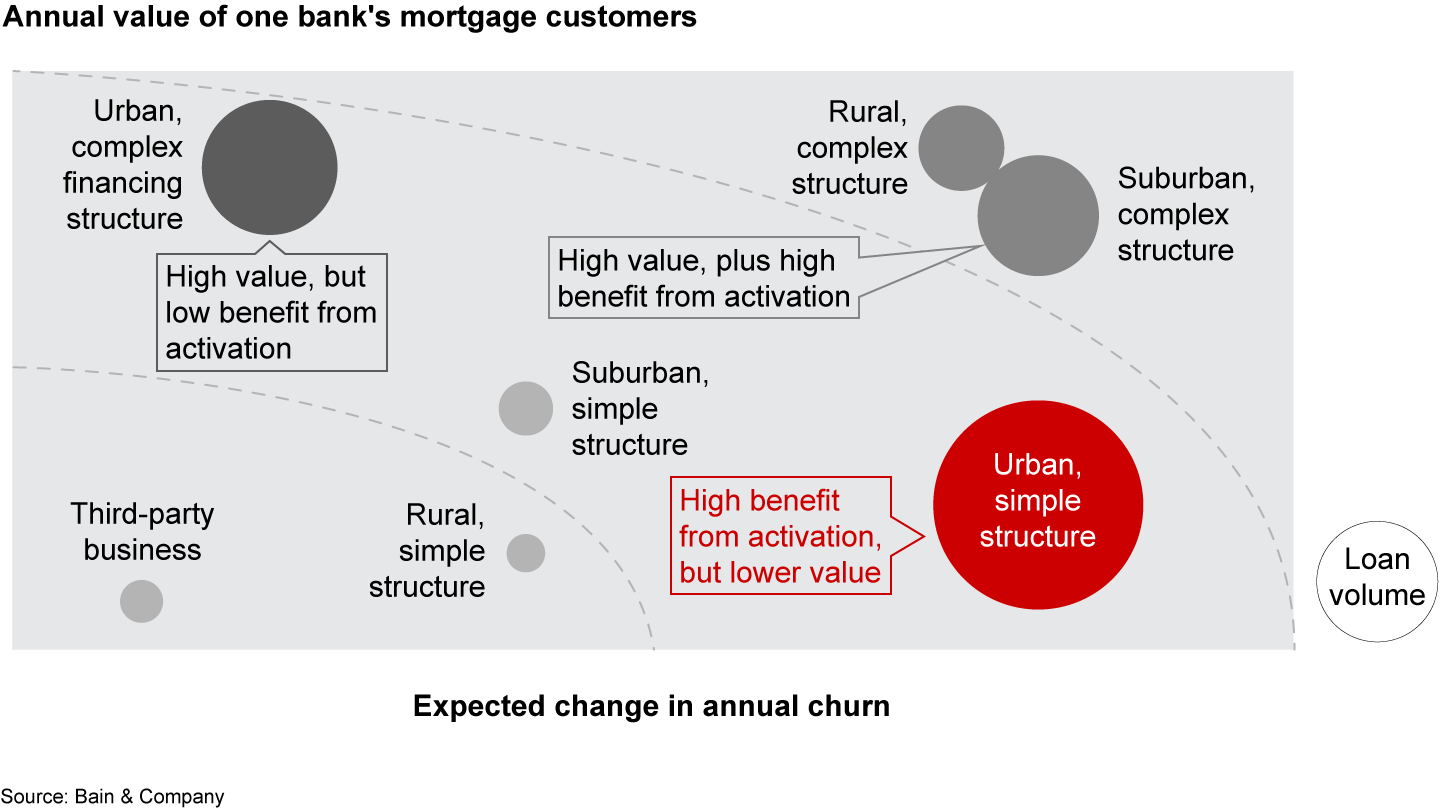
Metrics of advocacy such as Net Promoter Score, channel usage, brand preferences, and others can help derive useful clusters. These metrics come from internal and external data sources, starting with what is readily available and evolving through further collection of select data.
One Italian bank uses advanced analytics to automate client diagnostics in its lending to small and medium-size enterprises, cutting client evaluation time by up to 60%. At the heart of this capability lies a proprietary credit engine capable of analyzing more than 100 features to cluster clients into micro-segments and also assess financial strength at the individual client level. Features range from a firm’s historical performance, industry forecasts, and peer comparisons to indicators of good corporate governance. Advanced analytics and machine learning have made such complexity tractable, mimicking the intuition of experienced lending officers and taking the credit business to new levels of efficiency and effectiveness.
Marketing technology embedded into software platforms such as SAP and Pega, using customer lifetime value metrics, can help identify target segments that are similar to the most profitable customers—boosting conversion rates by up to 20 percentage points. Statistical methods useful for granular segmentation include k-means clustering, latent class analysis, artificial neural networks, and random forests. Choosing the best method for a given situation typically depends on practical considerations such as data availability and quality, team skills and tools, and a company’s willingness to embrace dynamic segmentation.
Automate forecasts at scale, verified by expert judgment. Many aggregate forecasts produce unreliable numbers, in part because it’s hard to attribute the effect of an individual driver when a bank is rolling out multiple initiatives. A more effective tactic would be to decompose time series for credit demand, order flow, and other variables, and do proper baselining for each one.
Another hindrance to adoption at scale is relying on a manual approach, since it consumes so much time to collect and prepare the data, set up and tune the model, and then determine the right subsequent actions. Greater accuracy and practicality come from automating the forecasting process for a large number of subpopulations. Proven algorithms used by leading digital firms can tap the predictive power of a broader set of data sources, such as past revenues, macroeconomic variables, and Internet search trends.
A key part of forecasting at scale involves automatically incorporating the judgment of experts for a specific time series. Such expert forecasts include more information than statistical forecasts alone and adapt more gracefully to changing conditions or disruptive events. Having an analyst in the loop at scale requires automatic evaluation of forecast quality and intuitive visualization tools, as a Facebook technical paper points out. With too many forecasts for analysts to manually check each one, the system must be able to automatically identify those that may require expert intervention.
With these success factors in mind, Facebook developed Prophet, a procedure and open-source software library that enables more accurate prediction of cash flows across a variety of industries. According to Facebook back-testing analyses, forecasting error in a typical case using Prophet reaches roughly 20% over a 180-day period, much lower than the error rate of up to 70% from conventional ARIMA models popular with banks.
Predict loyalty accurately for the customers who don’t respond to surveys. Loyalty and advocacy remain essential to power a bank’s sustainable growth and economics. Customers who are promoters spend more with their bank, cost less to serve, and are more likely to refer friends and colleagues, when compared with detractors.
These days, gauging loyalty has become trickier. Surveys typically reach only a fraction of customers, at great expense, and survey overload has forced companies in many industries to obtain insights into customers’ perceptions of the brand and different episodes through alternative means.
One viable alternative is to train a predictive model on questions of advocacy and churn, using a data set with known drivers of Net Promoter Score. A predictive model starts with basic features present in ordinary segmentation, such as channel usage, the frequency and nature of sales and service interactions, product usage, and revenue. It then taps more advanced sources of data, such as natural language processing of contact center conversations, including volume and tone.
Social network analysis can also be useful here. Constructing a social graph for the target customer segment, based on households, institutional relationships, or transaction data, will produce a measure that captures the overall connectedness of an individual customer. Innovators like Latin American online lender Kueski use credit history and social graphs combined with other online information to decide credit approval within minutes. One telecommunications firm has validated connectedness as a key factor in churn: More and stronger connections reduce churn from 0.8% to 0.6% per month, whereas fewer and weaker connections increase churn to 1.1% per month (see Figure 2).
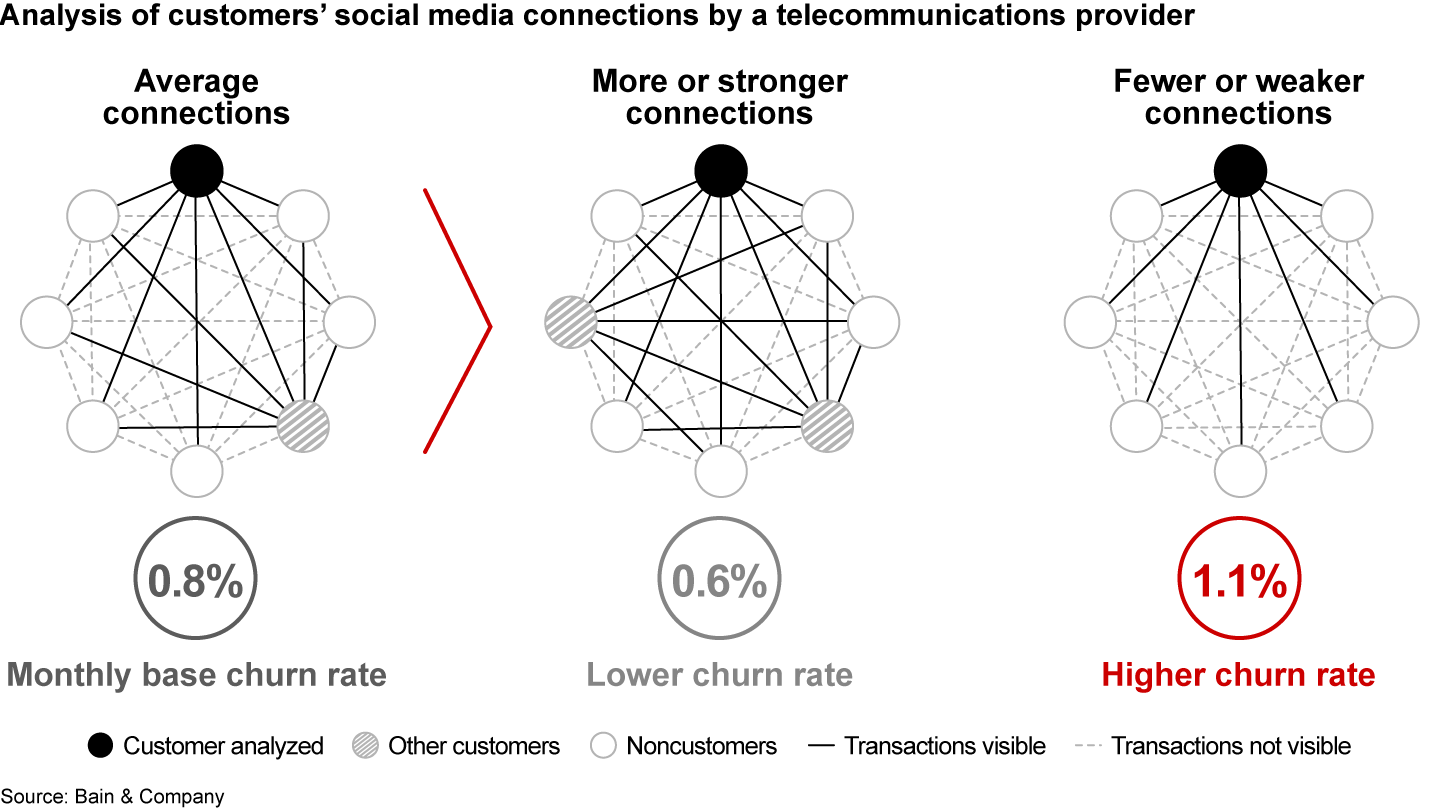
Once up and running, predictive NPS® guides employees to take the best next actions that will help earn loyalty, such as automatically matching the right contact center agent to a customer calling with a particular problem. For example, one bank using a predictive model achieves 70% predictive accuracy and a 30% success rate on commercial campaigns, compared with a roughly 5% success rate for the average campaign (see Figure 3).
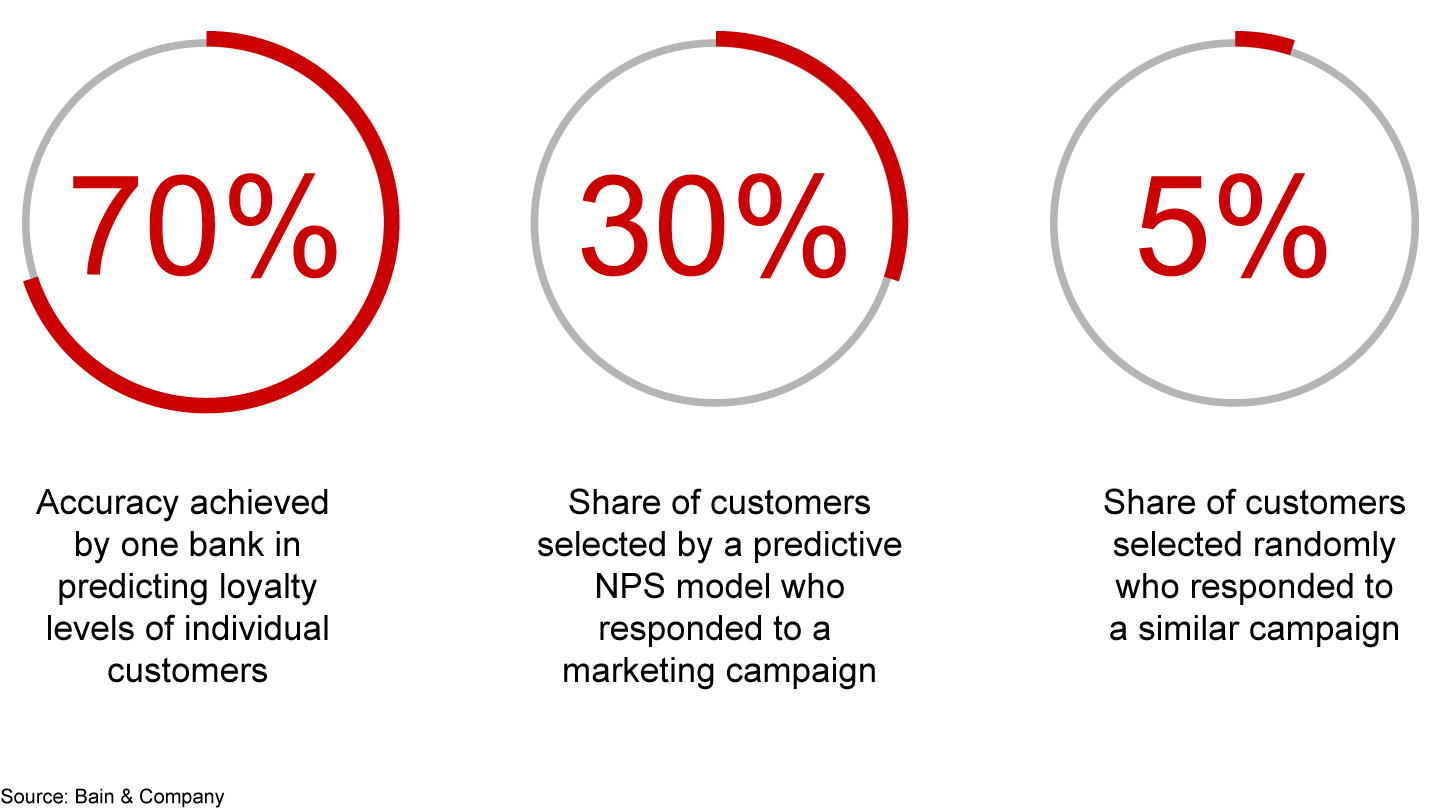
Understand what really causes customer churn—and what realistic steps will reduce it. Traditional bank churn models provide a single aggregate number based on customers’ propensity to churn, which is not useful to employees on the front line. It’s unclear what factors account for the number, what action to take, or how to fix root causes.
Predictions with no practical recommendations are all but useless. Explainable artificial intelligence (XAI) can change the game in this regard, by revealing the relative importance of different variables on an individual’s likelihood to churn. Capital One, for instance, uses XAI to ensure that humans oversee every decision that goes into the AI model, leading to fair, unbiased, and explainable outcomes.
Banks can augment their models by asking departing customers why they left. Once a bank knows the importance of each factor in causing churn, it can devise specific actions to deal with them. Frontline teams must be equipped with the right tools to execute the best actions, whether those are tailored offers, sales material, call center scripts, or something else. Those steps will be continually refined based on fresh data and experience over time.
Rapidly test and learn which offerings resonate with customers. Of course, a bank will rarely know right off which actions will work and which won’t. This is where continual experimentation plays a role.
Successful digital natives such as Booking.com and Expedia run thousands of digital experiments at once, involving millions of users. However, when Stefan Thomke of Harvard Business School studied data on experiments from more than 1,000 companies, he found that many run no more than a few dozen that have little impact. The central reason, Thomke found, is culture. In the eyes of many organizations that emphasize efficiency, predictability, and “winning,” the many experiments that by their nature do not succeed are viewed as wasteful—when, in reality, these experiments are essential for keeping in tune with the market.
Regardless of their current challenges, incumbent banks can benefit. They can use open-source or third-party tools from providers such as Optimizely for analytics and customer engagement. They can develop testable hypotheses on best next actions along a multitude of choices—and agree on meaningful metrics to consistently retain and activate customers (see Figure 4).
Adopting a granular, end-to-end perspective on conversion has been shown to be most effective in generating immediate results. Relevant metrics for converting prospects in the funnel might include the share of customers who open an email, click on the link in that email, receive advice, make a purchase, and stay on for at least a year. Adept testers will home in on suitable measures to boost conversion at each stage, while keeping in mind the broader challenge of customer experience: What is the most seamless way to help customers accomplish their fundamental objectives?
A retail bank in Indonesia that faced declining profitability and a drop in high-quality customers used multivariate testing to improve its savings products and acquisition of customers in personal loans and credit cards. The tests identified the best offer design, resulting in a 50% improvement in operating profit through lower prices and a fivefold increase in the uptake rate for personal loans.
* * *
Banks and fintechs that shy away from data-driven retention and activation because of potential organizational, technological, or regulatory challenges will fall further behind in the competition for high-value customers. Why the urgency? Customers increasingly expect a personalized experience. While technology puts sizable benefits within reach, developing and effectively deploying the required skills takes time.
Moreover, Covid-19 and lockdowns have changed the macro environment and customer behavior in ways that make historical data less reliable. The crisis has put a premium on accurate forecasting and more personalized offers, as well as rapid testing and adaptation. After all, it is far more effective to activate current customers who are open to expanding the relationship than to seek new customers at any cost.

NPS Prism℠
Prioritize the customer interactions that matter most. NPS Prism is a unique customer experience benchmarking service that guides your creation of game-changing customer experiences. Learn more →
Net Promoter®, NPS®, and the NPS-related emoticons are registered trademarks of Bain & Company, Inc., Satmetrix Systems, Inc., and Fred Reichheld. Net Promoter Score℠ and Net Promoter System℠ are service marks of Bain & Company, Inc., Satmetrix Systems, Inc., and Fred Reichheld.
NPS Prism® is a registered trademark of Bain & Company, Inc.