論説
概要
- Generative AI is already improving productivity, but those gains haven’t yet turned into meaningful cost savings at some businesses.
- Three obstacles are blocking generative AI cost savings: lack of a day-one cost mission, insufficient internal sponsorship, and outdated end-to-end processes.
- Companies can overcome these barriers by pursuing a generative AI–powered cost transformation rooted in zero-based redesign.
Executive teams have become used to seeing ambitious targets for boosting productivity with generative AI. These targets are realistic. The first wave of generative AI deployment has only given a taste of its power to enhance the speed and quality of work, particularly in areas that were previously hard to automate, such as decision making, content creation, and complex data analysis.
In customer service, for instance, we see room for companies to reduce the amount of time an employee spends on manual responses by 20% to 50% (see Figure 1). In product development, it’s likely that software engineering will become 15% to 30% more productive, while HR teams should see a 40% drop in the time needed to write a job posting.
Generative AI’s dramatic potential to accelerate work is already starting to be realized
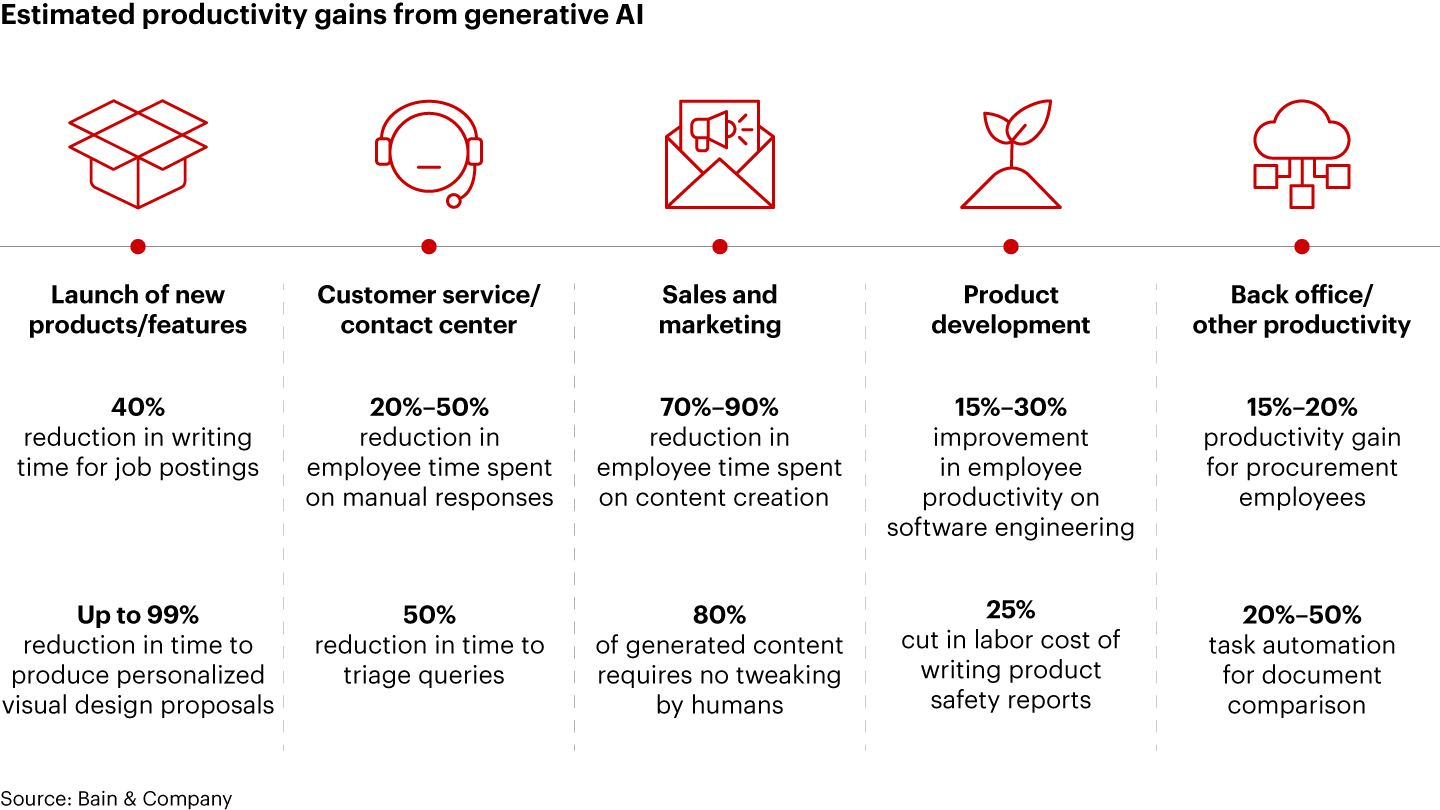

As they scale up their initial generative AI experimentation, many executive teams are receiving their own proof that the new technology will deliver on its productivity promise. Across functions, it’s clear that generative AI tools are helping employees get work done faster, sometimes even to a higher standard.
However, eager anticipation is giving way to a disconnect at a significant number of companies, across a range of industries. Executive teams and boards at these businesses are finding that generative AI productivity gains aren’t turning into meaningful cost savings, at least not yet.
Leading companies are on course to achieve cost savings of up to 25% by combining end-to-end process redesign with generative AI tool deployment, giving them more firepower to both expand their businesses and boost profits. At companies that are feeling the productivity-savings disconnect most keenly, it’s common to see savings of just 5% or less—limited gains normally associated with isolated experiments.
Failure to move beyond low-single-digit savings is putting affected companies in a tough spot, especially if their investors are expecting to see early P&L impact from generative AI. Still, executive teams and boards that are in this position must resist the temptation to dial down their generative AI ambition, even if such a move would ease short-term pressure on profitability by reducing the sums they are investing in the new technology.
Instead, they need to gain a clear understanding of why the gap between productivity and savings is occurring. Then they need to find a strategic way to align generative AI productivity improvements with their cost management goals. The good news for executive teams is they can draw on a more familiar innovation to help with this strategic alignment challenge: zero-based redesign (ZBR).
Three barriers to generative AI cost savings
When generative AI burst into the mainstream, it was imperative that companies didn’t just sit back and watch. The technology was evolving so quickly and with such huge economic potential that executive teams had no choice but to start experimenting with it to avoid being left behind, perhaps forever.
More than a year on—with generative AI proving to be even more potent than we first imagined and with further upside in the cards—there’s no doubt that just getting started was indeed the right move in those early days. However, the rapid and often experimental nature of those initial responses has inadvertently helped to create three temporary barriers to realizing generative AI cost savings. We outline these barriers below.
Lack of a day-one cost mission. The most powerful performance improvement initiatives start with a clear cost mission. For the deployment of a new technology or similar cost transformation tool, that mission might be bound up in an ROI target and a clear timetable for achieving it, for instance. This sort of upfront commitment ensures that teams remain focused on the right outcomes, even if the size and timing of the expected savings might fluctuate as the plan progresses. Yet, in the rush to get a firsthand understanding of generative AI, it wasn’t always possible at the outset to pair use cases with a cost savings goal or find ways to hold teams accountable for converting higher productivity into lower costs.
Insufficient internal sponsorship. Generative AI execution requires a broad mix of expertise from technology teams and specialists in business units and specific functions. It also needs sponsorship from an executive leader who is responsible for taking out cost in the function. In our experience, that buy-in hasn’t always been happening, leading to situations in which technology and analytics teams circulate predictions of imminent multimillion-dollar cost savings—only to be met with bewilderment from a functional executive leader who should have been looped in from the outset.
Outdated end-to-end processes. Reducing cost is hard and does not happen organically—as a spontaneous by-product of experimentation, for example. It requires management to commit to a fundamental redesign of work that will often require an organizational overhaul, targeted headcount reduction, and change management.
These obstacles are causing some executive teams and boards to question the value of their continued commitment to and investment in generative AI, particularly as it relates to making their business more efficient. That concern is understandable, but it misses a solution hiding in plain sight.
Zero-based redesign for the generative AI era
To ensure that productivity gains turn into cost savings, companies need to change what work is done and how it is done. Time and again, zero-based redesign has proved itself to be a powerful tool for tackling both these tasks.
Unlike traditional cost-cutting measures that often involve across-the-board reductions, ZBR uses a company’s strategic priorities to fundamentally reassess and reconstruct business processes from the ground up. It radically simplifies end-to-end processes, removing complexity from high-value activities, eliminating or streamlining low-value activities, and removing duplicative work. Paired with generative AI, this approach becomes even more potent, enabling organizations to achieve transformative cost savings while enhancing operational effectiveness.
Generative AI is already creating new possibilities for the radical simplification that’s at the heart of ZBR. That doesn’t mean knee-jerk automation of entire processes, with all the inefficiencies they might contain in their current state. Instead, it’s about reimagining what each process should look like in the generative AI age—factoring in all the new tools that the technology is making available—and then retaining only the essential steps from those workflows.
Consider the manual data collection that goes into financial planning. Today, it’s likely that some of this manually collected data gets used, some not. Rather than rush blindly down the path of wholesale automation, a true generative AI–powered cost transformation would only automate collection of the data that matters, ceasing the unnecessary work altogether.
Across functions, generative AI is starting to amplify the abilities of human workers. In financial planning, for example, human professionals can now outsource the first draft of a forecast to AI tools that are geared to handling complex models more efficiently, allowing those expert workers to focus on the more value-added task of refining later drafts. Improvements such as these will increasingly expand a company’s capacity to get things done. So, once a generative AI–powered cost transformation has identified the work that’s necessary and how it should be done, the final step is to reset organizational capacity accordingly.
At leading companies, generative AI–powered cost transformation is already starting to deliver the deep cost savings of up to 25% that we identified earlier as an example of best practice. It’s also allowing some high-growth businesses to increase revenues faster than costs by limiting the accretion of new spending commitments as they push forward.
After disappointing results from one-off generative AI experiments, one wealth and asset manager is now pursuing $1 billion of annualized savings—about 20% of its entire cost base—identified in a cost transformation roadmap that combines end-to-end process redesign with generative AI tool deployment. In finance and compliance, for example, the combined approach is automating reporting and analysis, reducing workloads by more than 40%.
Crucially, executive teams can blend ZBR and generative AI without breaking stride. An achievable plan can be in place within six months in many cases, with as much as half the expected value realized within the first year. By better positioning businesses for a thriving future, cutting-edge cost transformation can also win over employees—even to the extent of creating a double-digit improvement in their willingness to recommend the company as a place to work, as measured by Net Promoter ScoreSM.
Questions to realign productivity and savings
It’s still possible to reconnect productivity momentum to cost savings goals, even though the urgent demands of generative AI experimentation have complicated that task. As a prelude to injecting the rigors of zero-based redesign, executive teams can benefit from frankly assessing their generative AI experiments and early scaling efforts.
They should then consider a few key questions about their progress so far, including:
- Have we fundamentally rethought how efficient workflows and processes can be in light of generative AI’s transformative potential?
- Do we have a path to translating generative AI experiments into scale efficiencies and tangible financial return on investment?
- Do we have a clear perspective on how our organization will evolve as generative AI’s capabilities rapidly advance?
The answers to these questions can be a starting point for realizing the full power of generative AI and once more proving the equation that productivity can (and should) equal savings.