論説

概要
- Consumers and industries are adopting innovations at a much faster pace than years ago.
- Anticipating important innovations ahead of the competition can significantly increase growth and shareholder value.
- Four forecasting tools used in combination are more effective than any one alone at predicting the tipping point for new technologies.
- Leading companies have started using this approach to win first-mover advantage.
It’s not easy to peer into the future and spot emerging technologies or innovations that could reshape an entire industry. But what if some companies could see around the corner better than others? For executive teams and boards of directors, the ability to predict if, and how fast, new technologies will take off would be invaluable.
A handful of utilities and oil and gas companies, for example, realized that fracking technology would disrupt the industry long before analysts and the rest of the world started talking about that shift. They were watching for a critical signal that fracking would become financially viable, and it came in 2007, three years ahead of the shale gas boom. Anticipating a pivotal shift in the market, these companies positioned themselves to take advantage of the shale revolution.
New technologies often prompt a vision of sweeping change long before we know if they are viable, or how many years it will take to bring them to market. Scientists, economists and other experts foresee robots taking over jobs in service industries; genetic engineering revolutionizing agriculture; lab-grown meat supplanting the animal protein industry; autonomous vehicles becoming the new mode of transportation, with fleets of driverless cars replacing individual vehicle ownership and eliminating millions of truck driving jobs; and the Internet of Things revolutionizing manufacturing. Each of these innovations looks capable of dramatically altering whole industries. And some technologies, such as those that create platforms, can disrupt markets far beyond traditional industry boundaries.
So it’s a rare executive or board that hasn’t struggled with the question of when to bet on new technologies. Consumers and industries adopt innovations at a much faster pace today than years ago, and expert forecasts often fall short. In 2017, the price of battery packs for electric vehicles, for example, fell under the level analysts had previously forecast for 2020 (see Figure 1).
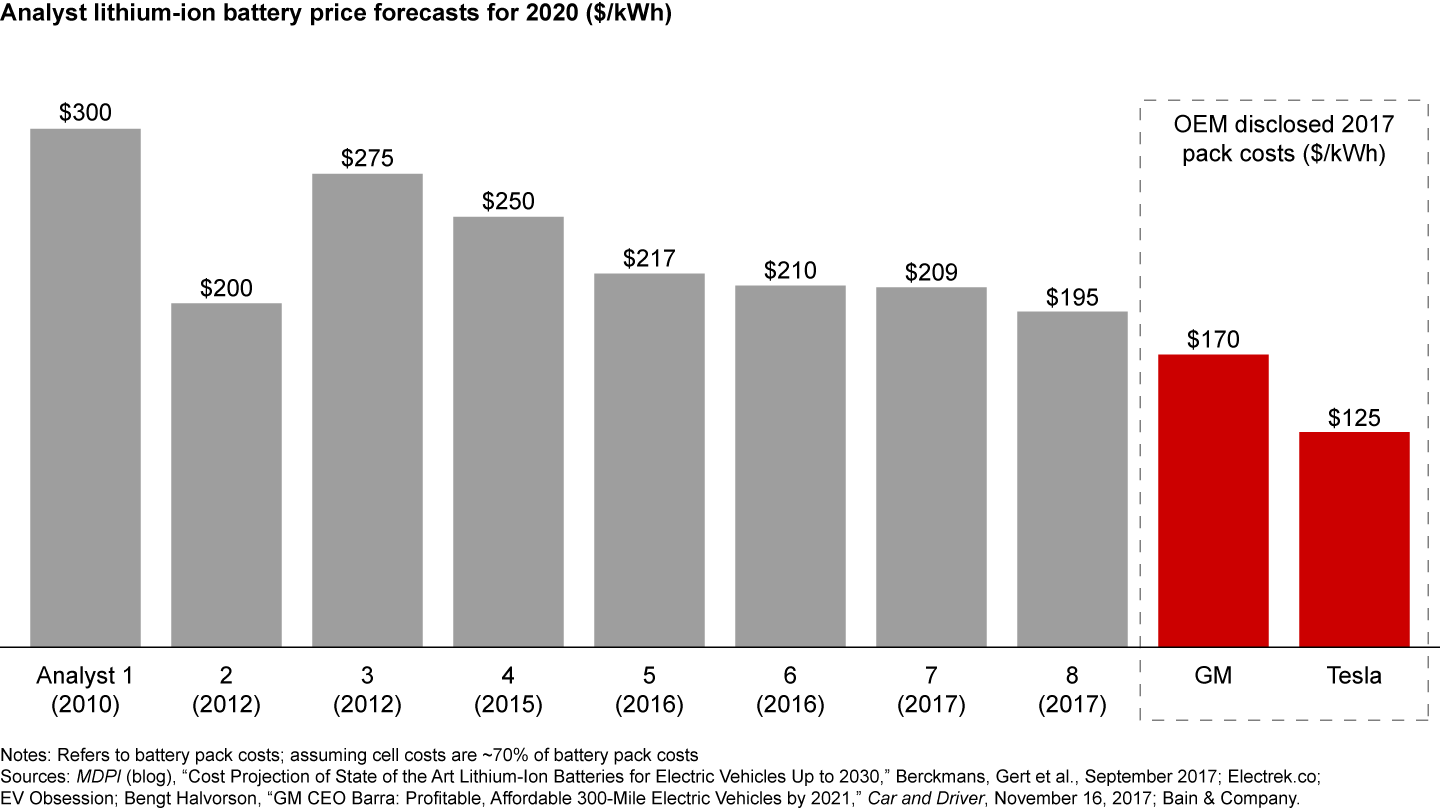

Companies that fail to anticipate or adapt to new technologies risk being blindsided by a sudden shift in market perceptions and falling valuations. The reason: Investors react faster than consumers to potential disruption. In the four years following Apple’s release of the iPhone in 2007, BlackBerry producer Research in Motion lost 90% of its market valuation, even though revenues more than tripled over the same period.
By contrast, leadership teams that take a more analytical approach to what’s coming around the tech bend gain a first-mover advantage. The ability to react even a few months ahead of the competition can be worth millions or billions of dollars. In the shared mobility sector, Uber secured a strong lead by being first to market and three years ahead of No. 2 Lyft. In 2018 Uber had $50 billion in bookings compared with $8 billion for Lyft. As of this writing, Uber’s valuation of around $70 billion is nearly three times higher than Lyft’s IPO value.
Netflix won a similar first-mover advantage when it launched video-on-demand service in 2007. By rapidly anticipating consumer demand for Internet-based streaming of movies, Netflix not only precipitated the demise of Blockbuster, which at that point had a market capitalization of $1.5 billion, but also beat rival Hulu to the market by a year. Today Netflix has a valuation roughly 10 times greater than Hulu’s, demonstrating just how valuable it can be to be first to market when responding to disruptive trends.
Predicting tipping points: A new approach
For decades, company leaders, heads of strategy, technology development groups and other industry experts have deployed different forecasting tools and economic models to gain an edge in predicting change. Each tool is useful in specific settings, providing companies with insights that helped guide strategic actions or investments—and each has its limitations. What’s been interesting over the years is the way successive tools filled in some of the gaps. There is no crystal ball, but we’ve seen some patterns recently that point to an intriguing and provocative question: Could combining some of these tools result in a sum that is greater than the parts? Would an artful blending of insights give leadership teams a jump on the competition in adjusting strategy?
In our experience, four forecasting tools, used together, can help predict whether a new technology will take off, when the tipping point will arrive and what the speed of market adoption will be. The four are: experience curves (e-curves), which show how unit costs decline relative to increased production volume; Elements of Value® analysis, to identify attributes customers value most in a product, such as reduced cost or saving time; adoption curves (s-curves), which forecast the likely pace of adoption and saturation point; and analysis of barriers and accelerators, such as government policy, technology or consumer attitudes that could slow or speed the arrival and penetration of an innovation.
It may sound complex, but tipping points analysis is a logical set of steps that can build a fuller picture of the future, much like putting together the pieces of a puzzle. The leadership teams that have used this combination of lenses know that it won’t produce an algorithm to predict the exact timing or speed of the next disruption. What it does provide is a set of insights around which executive teams can construct the most likely scenarios, understand the variables that matter most, and monitor them to help decide when to act. Leading companies have used these tools to anticipate important innovations as much as three years ahead of widespread market adoption, significantly increasing growth and shareholder value.
Let’s walk through the sequence of events that convinced us to combine these four tools into the tipping points model instead of using them in isolation.
Bain Partner Mark Gottfredson discusses four tools that can help forecast disruption.
The experience curve
Historically, industries have used experience curves (e-curves), to understand the pace at which production costs will fall. Specifically, e-curves plot the learning rate for bringing down the cost of production. The slope of the curve shows how unit cost declines as production experience grows.
Leadership teams rely on e-curves to set targets for cost reduction over time—and to predict when the cost of new technologies is likely to decline to a level that unleashes consumer demand. For example, Moore’s Law is an approximation of the experience curve for computer processing power and cost. Using it, companies have set targets for what their products will have to cost to compete in the future.
Over the years, we have found the e-curve to be a powerful tool. Rational consumers are likely to adopt a new innovation when the price matches their perception of value. So companies track the declining cost of production to anticipate the moment when market demand will take off. The tipping point is the threshold price at which people decide to switch to an innovative new product.
The alternative approach—classic cost-modeling methodology—takes a more simplistic view of cost movements. As a result, it often fails to predict accurately the speed at which costs decline. E-curves, which include the effect of accumulated production experience, economies of scale and technological advancements, are a more useful tool in forecasting when a new innovation will reach cost parity with an existing product.
Take the case of electric vehicles. At present, the price of electric vehicles is prohibitive for most consumers largely because of the high cost of lithium-ion battery packs. So the battery pack e-curve offers valuable insights about when the market could reach a tipping point for mass consumer adoption of electric vehicles.
Experts believe demand will take off when the cost to produce an electric car and an internal combustion vehicle are the same. That would require the price of battery packs to fall to about $100 per kWh or less. Our e-curve analysis, which correctly forecasts the current battery pack price, shows the cost of production should reach that tipping point by 2020–23, two to five years earlier than the projections of analysts relying on traditional cost modeling (see Figure 2). As we noted above, analysts’ forecasts based on traditional cost models have not kept pace with declining prices because they tend to calculate costs from a bottom-up perspective.
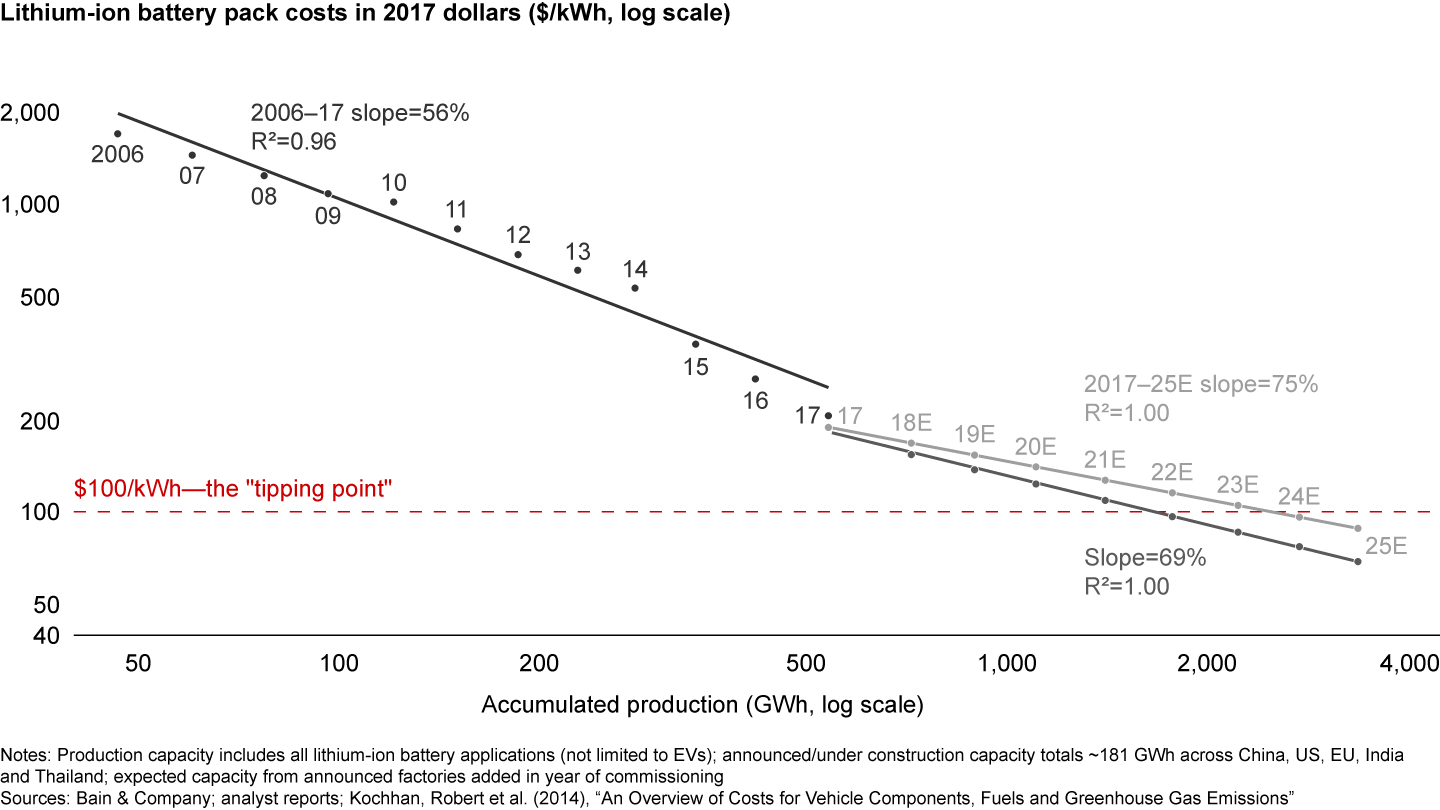

E-curve analysis can provide companies with valuable insights about declining prices and anticipate when breakthroughs may occur. But they do not predict which specific technological innovations will push costs down. There are thousands of engineers trying to bring those costs down, and no analyst can capture all of that innovation. Consequently, by 2017, battery pack costs already had dropped below the level of analysts’ forecast for 2020.
The experience curve has its origins in manufacturing and is an effective tool for predicting tipping points in industries where cost-per-unit typically is the main cause of disruption. But with some products, cost is not the primary factor determining a customer’s decision. Instead, it’s increased value. The price of new car models tends to rise from year to year because they incorporate the latest technologies and offer improved quality, fuel efficiency and safety.
Cloud computing is another example where improved capabilities, not price, propel consumer demand. Between 2014 and 2017, the headline price of cloud services did not decline much, but the amount of storage capacity, price per gigabit of RAM, average utilization per hour and the overall network performance all increased at an accelerating pace. As a result, the total price of the service declined significantly. The downward slope of the e-curve on a capability-adjusted price persuaded many companies to shift to cloud-based services. Cloud technologies also enabled and accelerated the initial growth of new services such as the messaging app Snapchat, which in turn spurred the growth of the cloud.
Companies that understand what consumers value most can use e-curves in a more sophisticated manner. Take the evolution of music players from vinyl records to streaming services. The price didn’t drop much from one generation of technology to the next, but functionality improved significantly. Cassette players gave consumers the ability to record music at home and provided greater portability. The Walkman offered music on the go in any location. Compact disc players improved listening quality and gave consumers the freedom to skip tracks of music. MP3 players reduced the size of music systems and increased capacity to store music while introducing the ability to create individual playlists. Finally, music streaming services delivered unlimited music at reduced cost (see Figure 3).
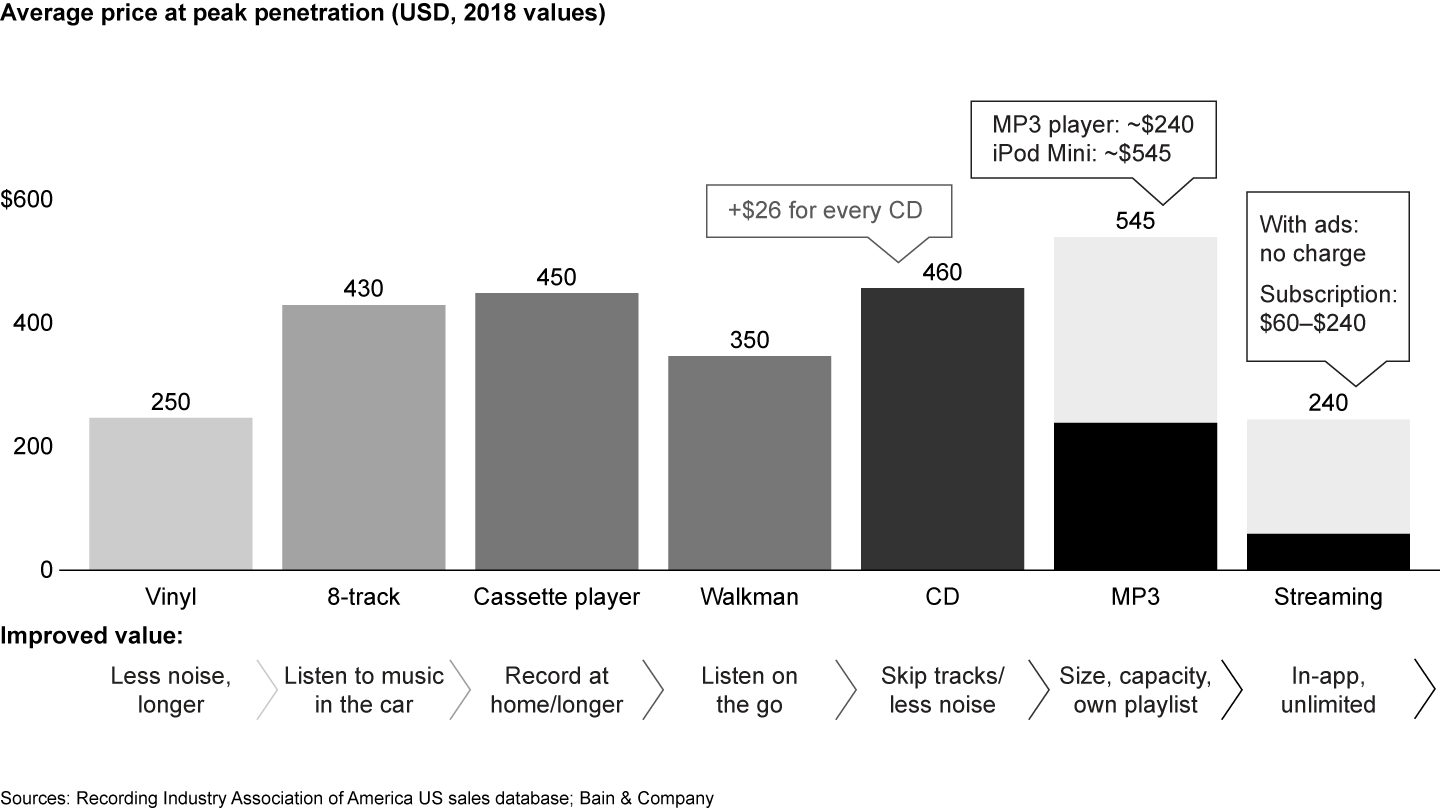

The companies that bet on the winning technologies in the evolution of music players did not try to bring prices down. Instead, they added features or convenience that customers valued, prompting them to switch.
As we worked with e-curves over the years, it became increasingly clear that to more accurately anticipate technological change, we needed to better understand the value that features and qualities bring to consumers, as in the music player example.
The Elements of Value
Consumers may switch to an innovative new product—even when the price is higher than the original—if they perceive that the new product offers greater value. The added value may be a quantum leap in quality or features that improve ease of use.
As part of his research on factors affecting customer choices, our colleague Eric Almquist in 2016 identified 30 Elements of Value and developed an analytical model around them. These elements address four types of value that consumers experience in a product: functional, emotional, life changing and social impact. Our model traces its conceptual roots to Abraham Maslow’s hierarchy of needs. Maslow argued that human actions arise from an innate desire to fulfill needs ranging from the very basic (security, warmth, food) to the complex (self-esteem, altruism). Bain’s Elements of Value pyramid expands on Maslow’s insights by focusing on people as consumers and describing how they experience the value offered by products and services.
The elements that matter most to consumers help explain why they prefer one product over another. Functional elements such as reducing cost, improving quality, saving time and simplifying a product make up the base of the value pyramid. The next level involves emotional elements, including design and aesthetics, wellness, fun and entertainment. Above that are life-changing elements, such as affiliation and belonging, motivation and self-actualization. The top of the pyramid is self-transcendence.

The B2C Elements of Value
Knowing what consumers value can help companies improve performance—and even unlock new markets.
The introduction of the Apple iPhone in 2007 highlights how these Elements of Value can turn an industry on its head. Compared with its feature phone predecessors from BlackBerry and Nokia, Apple’s first iPhone was more expensive, but it offered customers an entirely new combination of values by integrating the features of a cell phone, browser and music player all in one. The iPhone interface was simpler and more integrated than that of feature phones, and its design was more user-friendly. It ushered in the era of the smartphone, rapidly winning over consumers and dooming products that did not offer the same set of values. Within four years of the iPhone’s introduction, smartphones dominated the market, and within six years feature phones had nearly disappeared.
Similarly, Amazon’s e-commerce model disrupted the retail landscape by addressing consumers’ unmet basic needs. Customers saw value, for example, in online purchases as faster, easier and lower cost than in a physical store. They also appreciated the variety of offerings, quality and saving time. Amazon then created Amazon Prime and has increased the value of that service on multiple dimensions every year since (see Figure 4). The value that is created differs by type of product and has therefore led to different rates of penetration for different types of products.
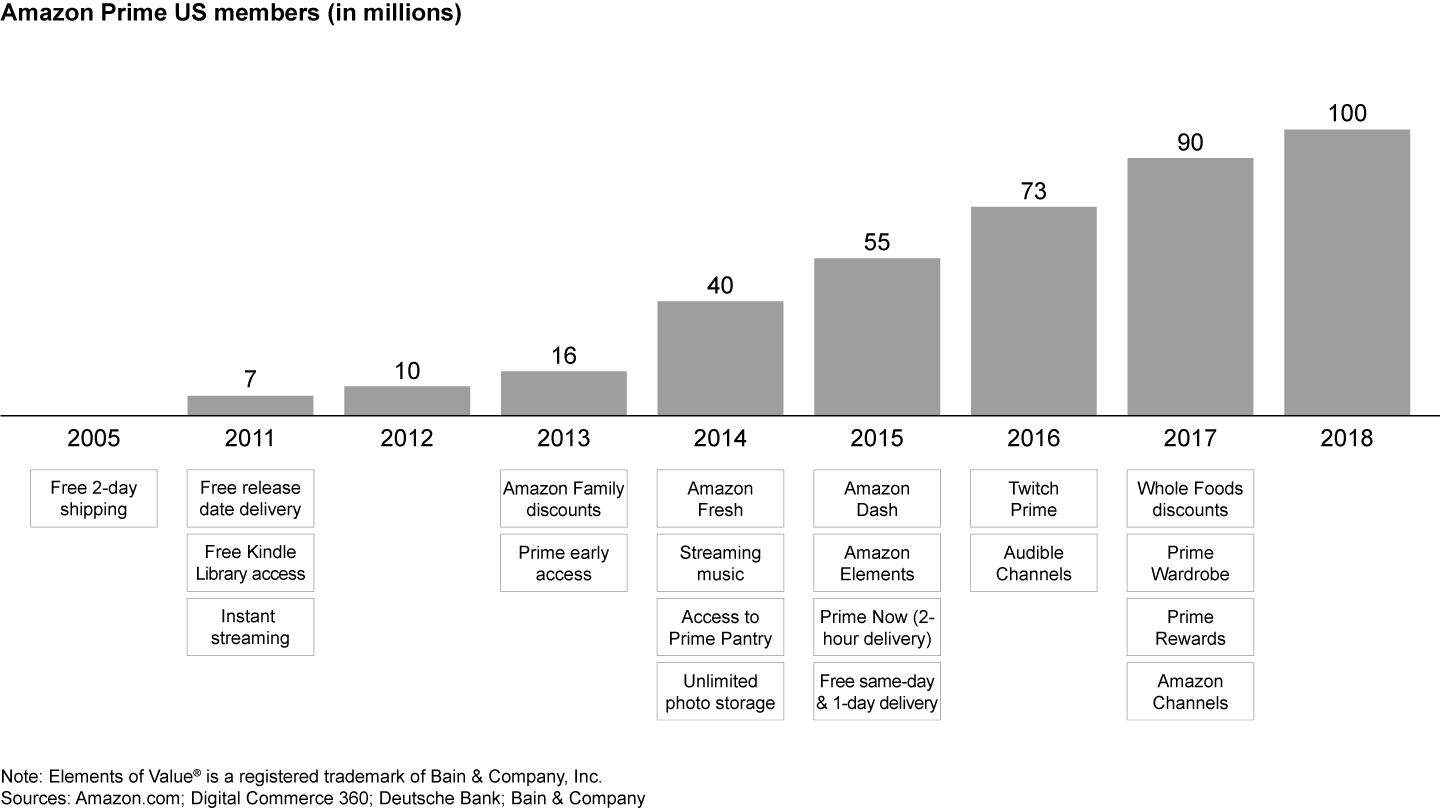

An analysis of the elements that customers value most can help companies more accurately predict when new technologies will take off.
Specifically, companies can use conjoint analysis to place an effective dollar value on each element of value for specific new technologies.
For example, in research conducted with Dynata, we found that electric vehicle owners significantly value complex factors such as ongoing cost savings, design and aesthetics, as well as the sense of belonging that consumers get from an electric vehicle. Together these higher-level factors contributed to an increased willingness to adopt electric vehicles even when they cost on average roughly $4,000 more than a combustion engine vehicle. Additionally, when consumers received information about lesser-known electric-vehicle advantages, including low maintenance requirements, the number of respondents choosing an electric vehicle over an equivalent gasoline car jumped nearly 10%.
Bain & Company research shows electric vehicles score higher than traditional internal combustion engine vehicles on all Elements of Value. Electric vehicle owners, in fact, value four intangible and higher-order attributes, including hope, affiliation and belonging. Since consumers attribute higher-level values to electric vehicles beyond reducing cost, the tipping point could come sooner than our e-curve forecast for battery pack prices reaching $100 per kWh (see Figure 5).
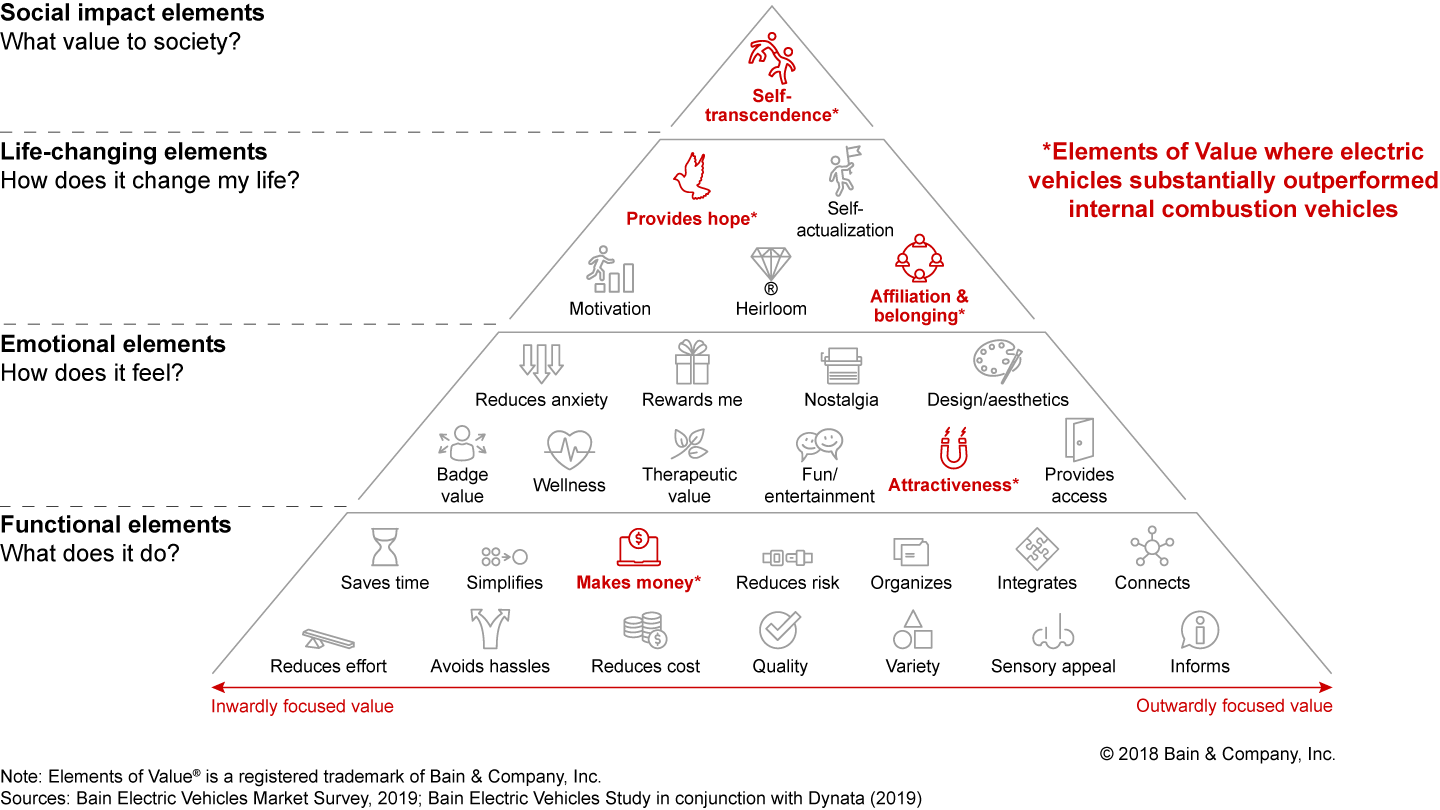

B2B Elements of Value
The Elements of Value pyramid is different for companies serving B2B markets, because the basic conditions for selling to business often are different than those for consumers. B2B markets leave less room for error and force competitors to perform at a minimum threshold level. Customer purchase experiences are more complex and less consistent. Reaching decision makers in a B2B business is also more complicated.
The common thread between B2C and B2B analysis is understanding what customers value. The speed at which companies adopt cloud services, for example, depends on the benefits of flexibility and scalability that the cloud offers over legacy hardware. For Netflix, switching to cloud services offered significant advantages, including a quick and easy ramp-up of computing resources on Amazon Web Services in peak periods. That process would have been more expensive if the company had invested in dedicated computer system resources. It also would have created excess capacity during periods of low demand.

The B2B Elements of Value
What matters to business customers? Subjective concerns often play a surprisingly large role.
Cloud computing already represents about 17% of the total IT market, based on public cloud revenues and spending on enabling infrastructure. Bain’s analysis indicates that market growth will continue at the current rate until cloud services reach the limit of the addressable market (saturation point) or a new IT paradigm, such as edge computing, disrupts the market.
As we started to understand the elements that consumers and businesses value most, we realized these insights were a powerful complement to e-curve analysis. They told us whether the product had value and whether consumers were likely to buy it. What they didn’t tell us, however, is the likely speed and extent of adoption. The tool that addresses these factors is the adoption curve (s-curve).
The adoption curve
Traditional straight-line, bottom-up forecasts often underestimate how quickly consumers will adopt innovative new products, a rate that continues to accelerate. The average time from tipping point when sales take off to 80% of maximum adoption has decreased from about 18 years in the mid-20th century to about 8 years since 2000.
The adoption curve is a tool that predicts how rapidly consumers will switch to a new product by forecasting sales over time. That calculation is based on three assumptions: when the tipping point will occur, how fast different segments of the market will adopt a given technology, and the theoretical maximum level of adoption (see Figure 6).
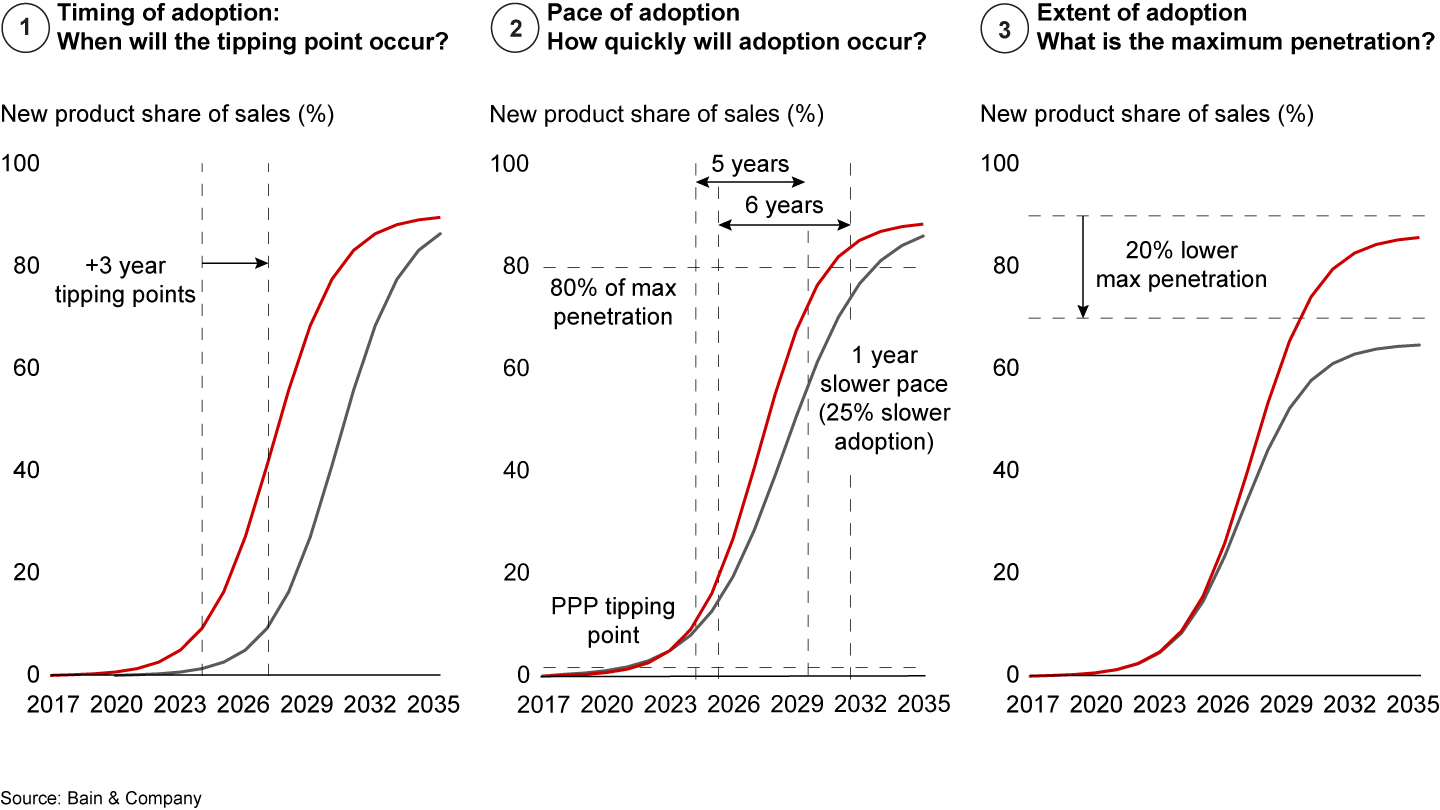

The tipping point for adoption occurs when a rational customer chooses the innovation over the existing product based on an assessment of three criteria: how well it meets functional needs, what additional value the new technology provides and whether the price is more attractive. In other words, customers will switch to a new product if its capabilities and price are more attractive than the original—insights which the e-curve and Elements of Value analysis provide.
However, the speed of adoption is likely to vary from one customer segment to another, based on the new product’s breadth of appeal to each group. So to forecast the rate of adoption, it’s important to define the key market segments and each group’s respective needs. An Elements of Value analysis can help companies identify different tipping points for each customer subgroup, creating a better overall forecast for the pace of adoption.
The tipping point and penetration rate of new services also depend on the value they provide. In e-commerce, for instance, the adoption curves vary for different products since some are better suited to e-commerce than others. Historically, consumers have adopted online offers for media such as film services significantly faster than personal care and beauty, because of price competition, wider selections, improved convenience and product trust, all of which are less important for categories like food and drink or personal care (see Figure 7).
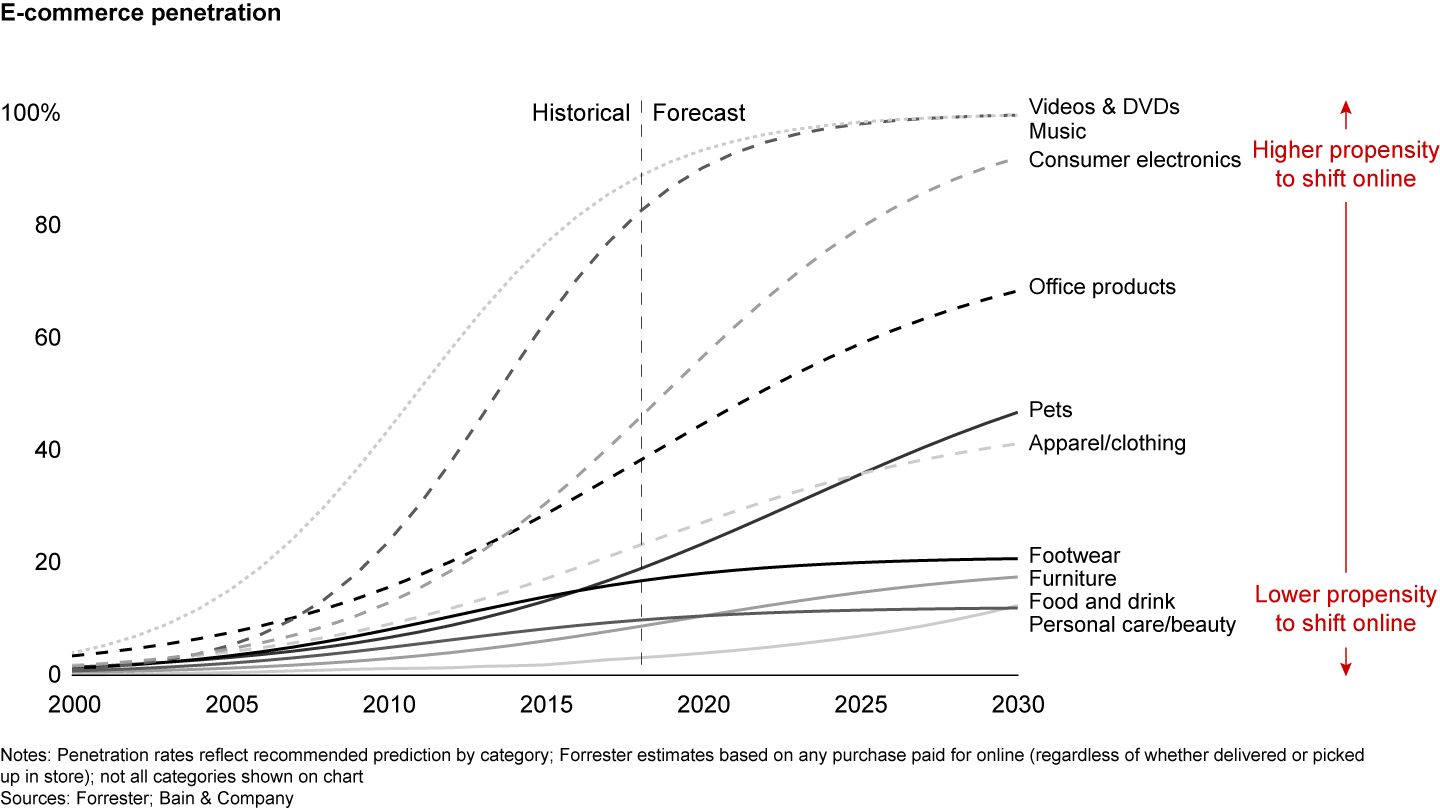

The first step in understanding how quickly consumers will embrace new technologies is plotting a curve based on historic penetration rates for comparable technologies. However, since many elements can affect the pace of adoption, it’s critical to adjust the curve based on accelerators and barriers. Without them, the risk increases that the forecast will be wrong.
The pace of adoption is also determined by how large a segment of the market is drawn to the Elements of Value the product provides. As we looked at the adoption curve for electric vehicles, for instance, we identified two main subgroups of consumers: individual vehicle owners and commercial drivers. Each group has different needs and uses for a car and, therefore, different perceptions of the value of an electric vehicle. As a result, the tipping point differs for each group.
Defining these subgroups allows us to create two distinct tipping points: one based on the economics for individuals and one for commercial drivers. Commercial enterprises such as taxi drivers and fleet owners are likely to switch to electric vehicles when the total cost of ownership is the same as for internal combustion engine vehicles—even if the purchase price is higher. Individuals are likely to buy electric vehicles when they become equally or less expensive to purchase than combustion engine vehicles (see Figure 8).
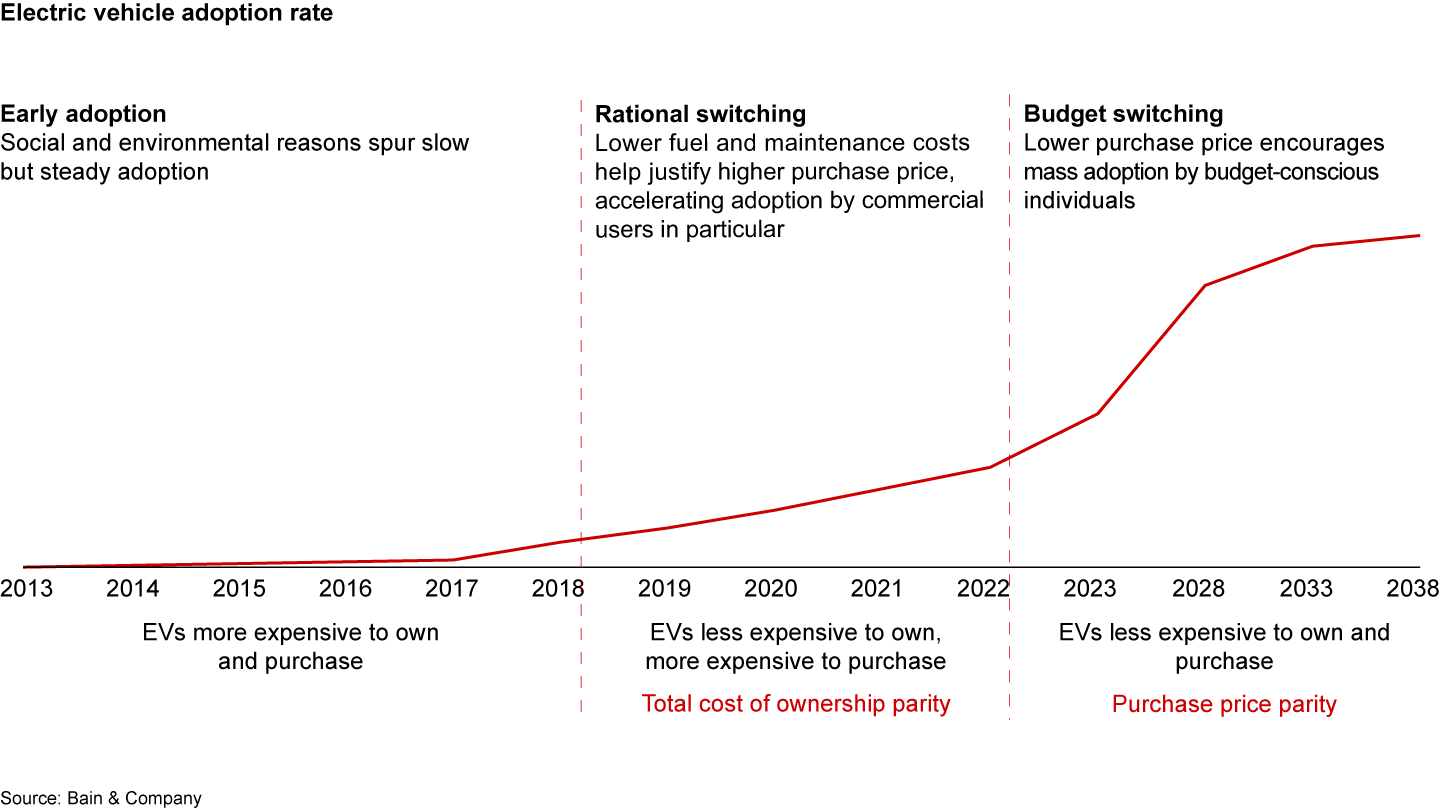

We have also used adoption curves in combination with survey data to increase the level of confidence in key assumptions. The resulting analysis provides a detailed view of how a technology is likely to take off under given circumstances. Returning to our previous example of battery pack prices, adoption curve analysis suggests electric vehicle sales could grow much faster than current forecasts (see Figure 9).
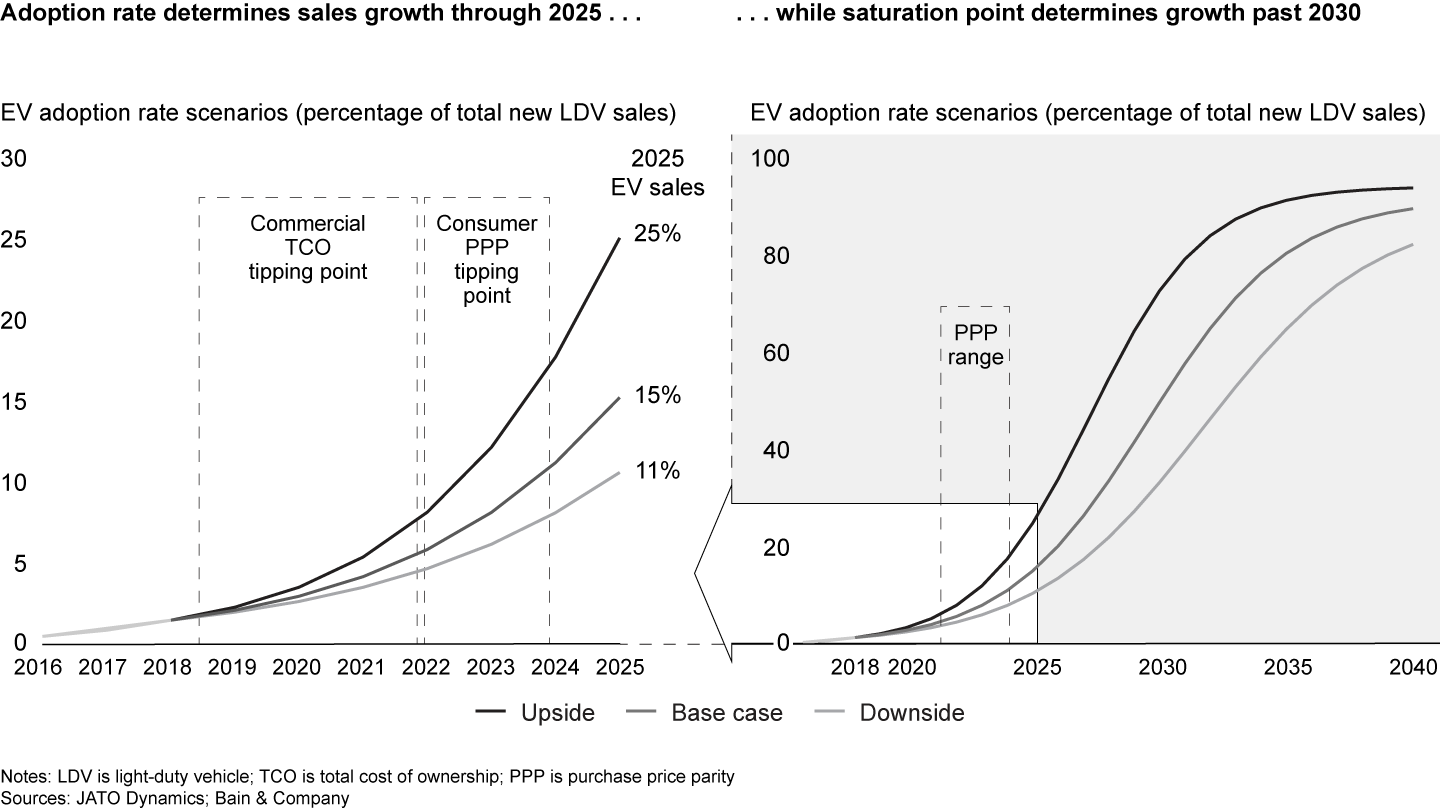

The adoption curve predicts how fast adoption is likely to occur, based on historical trends and assumptions about what consumers value. It adds valuable insights and perspective to our forecasting toolkit. But as we worked with adoption curves over time, it was clear that the tipping points model we were building still wasn’t complete. We needed to take into account external factors that can speed or slow technology adoption, such as government regulation or consumer attitudes toward a product.
Barriers and accelerators
In the fourth phase of our tipping points journey, we looked beyond economic models to other factors that could influence demand. By identifying industry-specific barriers or accelerators to new technologies, we were able to refine our projections and track developing scenarios with greater precision.
In particular, four structural elements—regulation, technology, economics and consumer behavior—can slow or speed adoption of innovative new products, altering the fundamental shape of the adoption curve, including the tipping point and the pace of adoption. Once we have a good understanding of these external factors, we use them as signposts to monitor the evolving pace of adoption. Identifying signposts is particularly useful when the timing of a technological tipping point is far from certain, and when a company is contemplating when to enter the market.
China’s 2007 decision to build large solar energy power plants and to purchase solar panels from low-cost producers was a key accelerator for the fledgling solar industry. The government’s investments pushed solar panels rapidly down the e-curve and sped market adoption. Importantly, China financed projects as opposed to inputs: It announced the volume of solar panels it planned to buy, creating a market, but purchased from low-cost manufacturers, creating a powerful incentive to reduce production costs. By contrast, the US government subsidized all solar-panel manufacturers, a policy that didn’t encourage companies to reduce costs. As a result, US subsidies failed to stimulate the industry’s takeoff.
On the other hand, government policy can also act as a barrier. Data privacy law, for example, may hinder the use of cloud computing in healthcare. Barriers and accelerators give companies a more robust and realistic perspective on the speed at which new technologies are likely to take off. Barriers to adoption of electric vehicles, for example, include infrastructure, limited battery manufacturing capacity, commodity bottlenecks, government regulations and battery pack costs. Each of these barriers is a valuable signpost for monitoring the pace of change.
With cloud computing, technology limitations remain a barrier to shifting additional work cases or workloads to the cloud, but innovation could enable faster adoption. Next-generation technologies such as containers and serverless computing also could accelerate adoption of cloud services by making it easier to develop cloud-native apps. And growth in artificial intelligence and machine learning may speed adoption with a new range of specialized solutions.
On the other side of the equation, the rise of edge computing could compete with cloud services. While cloud processing is centralized, edge computing pushes computing applications, data and services away from centralized nodes to the edge of a network to enable real-time analytics—a computing model particularly well suited to the Internet of Things.
Seeing around the corner
Leadership teams building a strategy in periods of uncertainty can fall into two traps. One is to decide on a likely future scenario and bet on it. Given the number of variables affecting the evolution of new technologies, that’s a rifle shot in the dark. The second most common mistake is betting on every square to manage risk.
Tipping points analysis helps companies avoid both those pitfalls by providing clearer visibility into likely future scenarios as well as the variables that could speed or slow change. That gives leadership teams more time to consider options and trade-offs and a head start on implementing and adjusting strategy.
What does that advantage look like? Utility CMS Energy used tipping points analysis to understand if and when fracking technology would become viable and push down natural gas prices. By tracking key signposts, the leadership team spotted the 2012 collapse in the price of natural gas three years ahead of time.
The company’s original long-term strategy had anticipated gas prices growing by 70% between 2010 and 2015. Fracking was a little-known technology at the time, but industry experts realized it had the potential to bring new sources of natural gas to market. In 2007, the technology looked promising, but the cost of fracking and government regulation made its viability and adoption highly uncertain.
Amid that uncertainty, the utility identified early indicators that fracking would take off, supply would grow and natural gas prices would drop. These signposts included e-curves on the cost of recovering gas, as well as substitution barriers and accelerators linked to regulatory and safety issues. Within months of setting up these indicators, the number of permits for fracking wells signaled that the new technology would take off. The increasing likelihood of a boom in shale gas production pointed to a collapse in gas prices.
Based on its tipping points analysis, the utility shut down all subscale coal units and announced plans to retire the rest. It bought a natural gas power plant at a fraction of the cost of building a new plant and restructured its gas supply contracts. Natural gas prices subsequently plunged 85%. The company’s strategy helped generate a compound shareholder return of 19% from 2011 to 2015, far above the industry average, and doubled the company’s market capitalization.
Tipping points analysis is not a perfect science. That’s why signposts are such a critical component in forecasting change, as the CMS Energy case shows. The forecasting tools we’ve highlighted are most effective when combined with a dynamic strategy and decision making.
A growing number of companies have figured that out, and are using tipping points analysis to anticipate technological change that could threaten their business models or create new opportunities. For these leaders, important signposts can deliver more powerful insights than months of detailed analysis. It also gives senior managers critical lead time to react first and adjust their strategies. In a heated race, every advantage matters. Companies that can see around the tech bend before their competition have the inside track.
Elements of Value® is a registered trademark of Bain & Company, Inc.
Mark Gottfredson is a partner with Bain & Company’s Performance Improvement and Advanced Manufacturing & Services practices, and he leads the firm's Automotive and Transportation Vehicles practice in the Americas. He is based in Bain’s Dallas office. Dunigan O’Keeffe is a partner and leads Bain’s Strategy practice in the Americas. He is based in the firm’s San Francisco office.
About Dynata
Dynata is one of the world’s leading single providers of first-party data contributed by people who opt in to member-based panels that the company manages and maintains. With a reach that encompasses more than 60 million people globally and an extensive library of individual profile attributes collected through surveys, Dynata provides precise, trustworthy quality data. The company has built innovative data services and solutions around this core asset to bring the voice of the individual to the entire marketing spectrum, from market research to marketing and advertising. Dynata serves nearly 6,000 market research agencies, media and advertising agencies, consulting and investment firms, and healthcare and corporate customers in North America, South America, Europe and Asia-Pacific. For more information, go to www.dynata.com.