Expert Commentary
Aftermarket sales is a crucial part of business for auto manufacturers. Selling spare parts and services can significantly boost both revenues and profit, since revenues per car can amount to several thousand dollars over a car’s life.
Yet car original equipment manufacturers (OEMs) and their dealers often capture only a portion of potential revenues. Historically, many of them lack transparency on the full potential of their sales and operations, even though they have vast data pools that could provide insights.
Advanced analytics offers an effective approach that provides reliable, data-driven insights to determine which aftermarket areas are performing well, and which need improvement. This commentary summarizes an analytical model that Bain & Company recently developed for a large auto manufacturer. The model addressed the following questions:
- What is the lifetime revenue potential of an individual car model for a specific aftermarket event or category, such as a brake change or 10,000-mile service?
- What is the annual revenue potential for a car model in a certain time range (say, from 6 to 8 years) or mileage range (from 35,000 to 60,000 miles)?
- Which aftermarket events drive revenue within these categories?
- Which events serve as triggers for cross-selling or upselling?
- What is the realized market share on these events?
The model’s logic
The analytical model computes full potential revenue and market shares based on various data sources provided by the OEM. The most relevant data includes anonymized invoices from the OEM’s service partners, prices and descriptions of existing spare parts, and car registrations per year for all relevant models.
The model features two innovations: identification of aftermarket events from millions of invoices using a customized market basket analysis algorithm, and the calculation of event probabilities over a car’s life, considering both the underlying number of cars as well as customer loyalty.
In this context, an aftermarket event corresponds to a combination of spare parts frequently seen on shop invoices. For example, the event “brake change” might contain the parts “brake disk,” “brake pad” and “screw,” belonging to the aftermarket category “wear.”
The logic of the model can be broken down into three steps.
1. Identify key events using market basket analysis. To boost revenues, car manufacturers want to target the most common aftermarket events, so they can raise their market share. Identifying common events can be challenging, since there are countless possible combinations of parts found on invoices.
To solve this issue, we applied an adapted version of the Apriori algorithm, commonly used for market basket analysis. Apriori is a form of association rule learning, which iteratively generates frequent sets of items from a long list, such as spare parts on an invoice. We developed a customized version of Apriori, one that considers not only frequencies of parts and part combinations, but also their prices (see Figure 1).
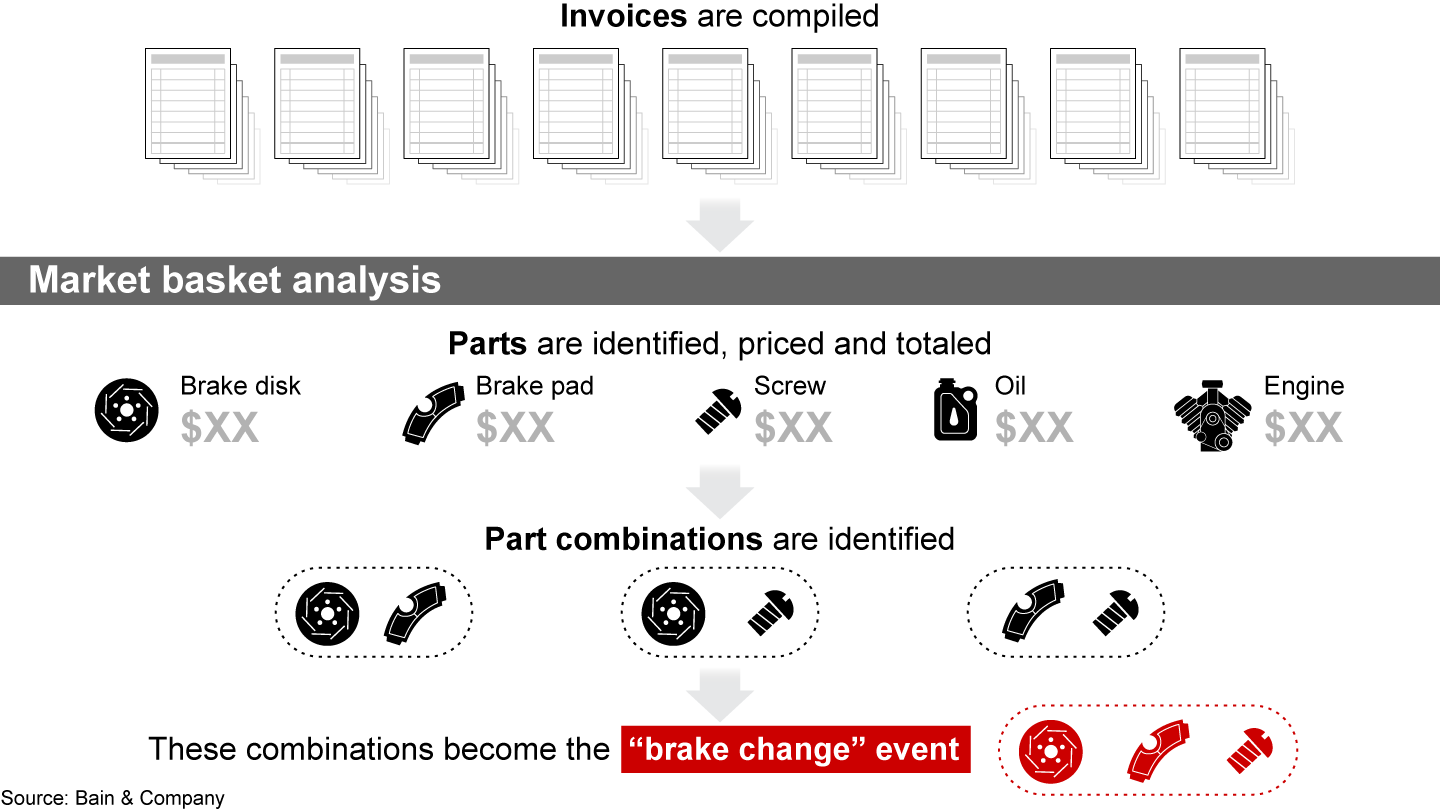

2. Compute the probability of events. After identifying relevant events, you can determine their occurrences over time by counting their presence on all available invoices. However, this renders only parts of the entire picture, and you will not be able to infer a car’s full revenue potential purely based on raw occurrence numbers, for two reasons:
- Car registration skewness. On invoices, you will see more younger cars than older ones, due to the scrap rate of older cars. To account for this age disparity, the raw event occurrences across all age segments have to be brought to the level of the first year of registrations, so that the event occurrences get adjusted to the same number of cars.
- Customer loyalty. Since many customers will bring their car to third-party shops, you need to apply a loyalty factor to the raw event occurrences. This factor indicates how many cars in a given age or mileage segment are visiting the OEM’s shops. To determine the factor, correct the number of invoices for the overall number of registrations for a specific age or mileage (see Figure 2).


By correcting for these aspects, you do not need data on nonloyal customers. You can compute the probability of a given event by scaling up the raw event occurrences.
3. Calculate the full potential and market share. We determined the full revenue potential per car by multiplying the event probabilities by the respective event price. In order to determine market shares, the full potential per car has to be scaled by the entire number of registrations. Hence, the market shares are the ratio of the actual revenue on invoices to the scaled full potential.
The model uses a dashboard to display the output—the revenue full potential and the market shares over different dimensions, such as aftermarket events and categories, age ranges or countries. Key users of these insights include the aftermarket strategy teams as well as the respective country representatives, who are responsible for steering overall aftermarket operations and sales processes.
To make this modeling as effective as possible, keep in mind the following principles.
- Accept only high-quality data. Reliable insights hinge of starting with a large, well-maintained database. Data quality will directly affect the quality of your model.
- Incorporate business knowledge. Experts in a particular slice of the aftermarket should participate throughout the entire development process, to provide domain-specific insights and validate results.
- Collaborate in cross-functional teams. Teams of data scientists, generalist consultants, and domain experts are essential for developing analytics solutions in a fast and agile fashion.
- Install reliable computing infrastructure. Complex pieces of model logic demand a reliable computing environment, in which cross-functional teams can iterate quickly over several model variations.
Advanced analytics techniques can allow any company to capture hidden insights from existing data pools. In the automotive aftermarket, this OEM gained more visibility into a key business area. Using a powerful, data-driven approach to analyze millions of invoices, through over 50 logical steps, the company:
- Gained deeper visibility into market performance (revenue full potential and current market shares);
- identified and mitigated gaps in customer loyalty; and
- tracked the effect of mitigation measures on loyalty.
Future expansions of the model might include the integration of additional data sources, such as vehicle telematics data or spending data from external auto shops (privacy regulations permitting), as well as personalized offers and discounts for customers based on their past behavior.