Brief
At a Glance
- Generative AI’s emerging leaders are avoiding protracted debates about the perfect use case for their companies.
- Getting started on both internal and customer-facing applications will accelerate learning.
- Leading companies are creating ethical and security guardrails around their AI strategy that empower, but don’t hinder, the organization.
OpenAI has opened executives’ eyes around the globe to what exciting things are possible with the newest evolution of artificial intelligence. As telecom executives digest the magnitude of how much large language models—and generative AI more broadly—could transform competitiveness in their industry, discussions about which AI use cases to prioritize have caught on like fire. In many boardrooms, long lists of potential AI investments are being drafted, debated, and agreed to, only to be deemed incomplete or outdated given the rapid pace of innovation.
These protracted debates are a waste of precious organizational energy. The use cases for generative AI—which uses sophisticated machine learning models to produce original text, images, and other content—in telecommunications are largely clear. Hence, we don’t think that our map of potential telco generative AI applications below (see Figure 2) provides any deep proprietary knowledge. Nor would we claim that no better list exists.
That’s just not the point. What is? Speedy action trumps perfect planning here. It’s more important for telcos to quickly launch an initial set of generative AI applications that fit the company’s strategy, and do so in a responsible way—or risk missing a window of opportunity in this fast-evolving sector.
Based on our experience working with companies worldwide on AI and other major technology initiatives, here are three guiding principles that can help executives make the most of their investments.
1. Stay away from AI “fever”
It’s important for executives to have a sense of what the technology can and cannot (yet) do, so they don’t get caught up in the hype and make misplaced bets. Many executives are already starting to recognize how generative AI can complement existing business capabilities to deliver greater value to customers, often in novel ways. Still, it’s early days for the technology, despite the promise it bears. Setting the bar too high will set up the team for failure as they work to develop and deliver the company’s first successes.
The simple and pragmatic solution is to apply “Horizon 1” and “Horizon 2” lenses (see Figure 1). This means prioritizing a first group of generative AI applications based on ease of implementation and risk level, then moving on to applications that are more complex and valuable. However, that doesn’t mean waiting until all the Horizon 1 applications have launched before building any Horizon 2 use cases. Nor does it mean that Horizon 1 use cases won’t require ongoing iteration and expansion. Without overwhelming the organization, getting multiple minimum viable products (MVPs) in the hands of customers and employees will expedite learning and accelerate transformational experiences.
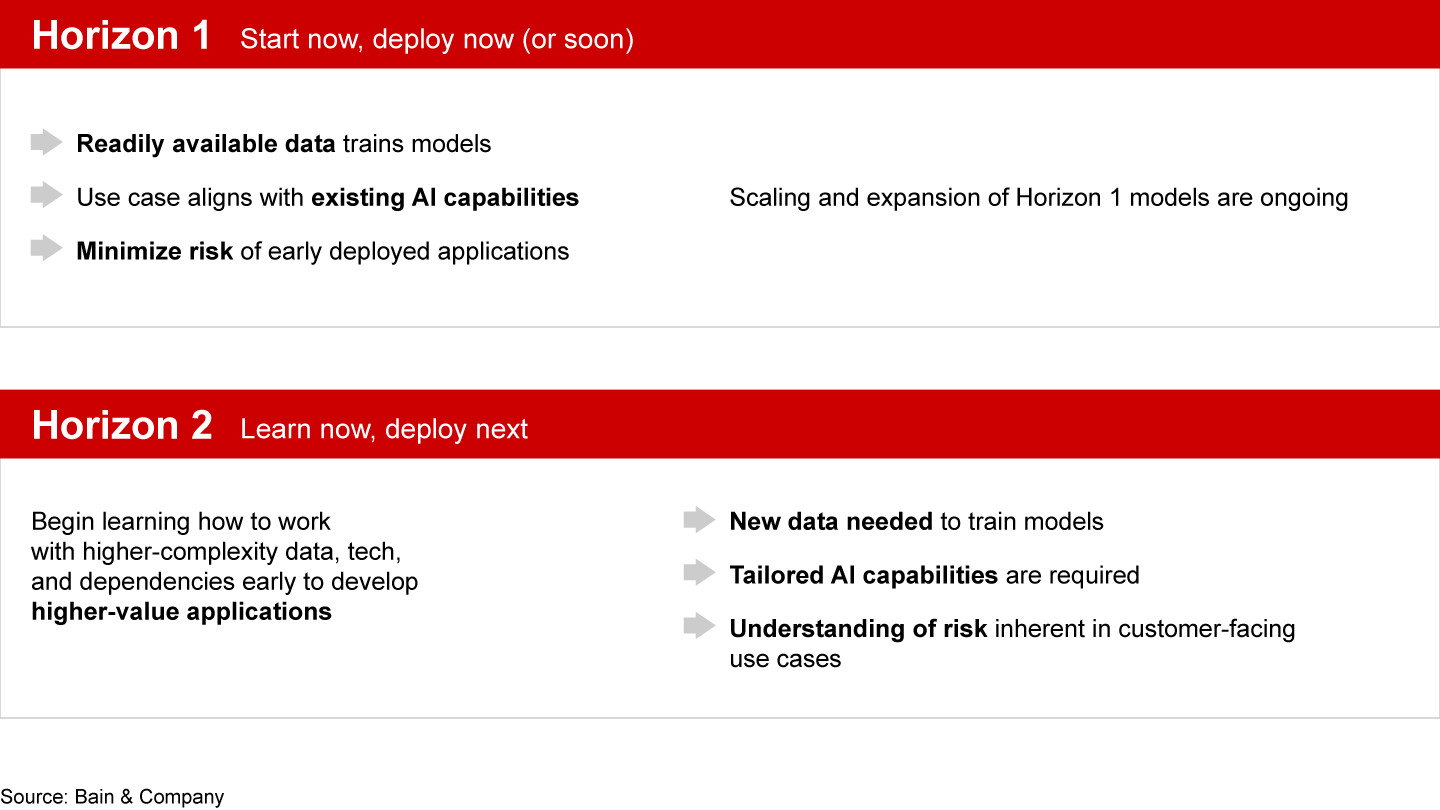

Applying generative AI to knowledge management is a no-regrets move under any circumstance for telcos (and all industries) because it can create immediate value across business functions. In telecommunications, one could imagine the application of that core use case across the organization: leveraging past creative assets for marketing campaigns, simplifying access to wireless plans and policies for contact center agents, or streamlining a long list of employee benefit-coverage questions for HR employees. Other Horizon 1 applications for telcos could include enabling more rapid (human-reviewed) testing and learning in marketing, automating routine code-generation efforts for the IT and data organizations, or quickly generating dynamic sales scripts for call center agents (see Figure 2). In Horizon 2 and beyond, the opportunities grow more sophisticated, whether it’s developing a real-time test and learn tool for marketing or an AI assistant for field technicians that can provide guidance in real time based on photos, voice cues, or text.
Identifying the right mix of use cases to pursue will of course differ for every telco based on its existing capabilities, budgets, and strategy. For example, one European telco has started to invest in prototypes to help its graphics team create marketing assets faster, and it’s exploring a coaching app for its customer service agents to better understand past customer behavior and activities in order to make more personalized recommendations.
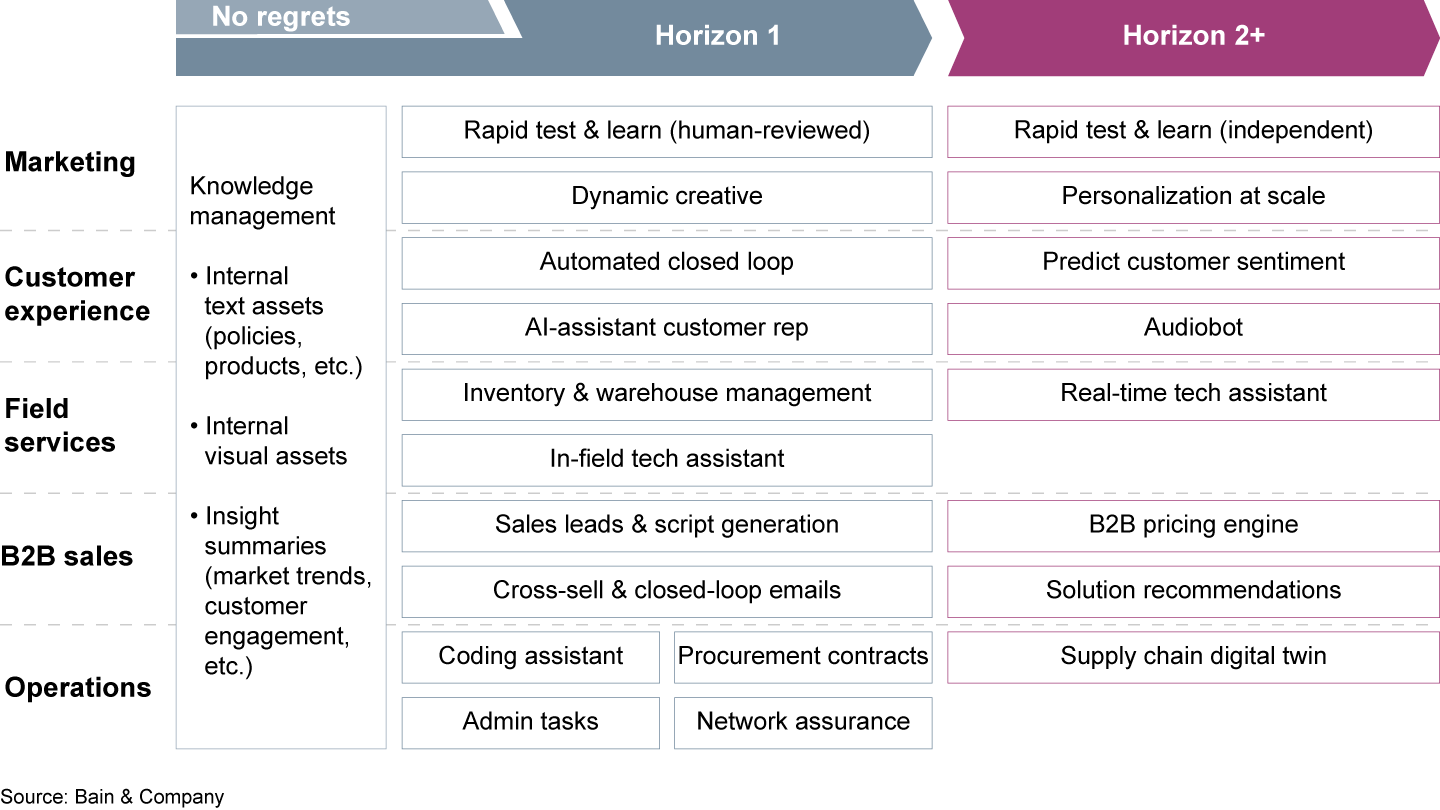

2. Avoid science experiment syndrome
Just as seeking the “perfect” generative AI use case can waste scarce time and resources, so too would adopting a perfectionist attitude toward the development of AI applications. Given telcos’ complex and mature tech stacks, it’s understandable that executives may want to spend ample time vetting generative AI applications before putting them in the wild and investing in scaling them up, or thinking hard about which core technology or start-up partners to pursue. But overweighting those considerations risks trapping generative AI applications in the proof-of-concept phase, never to deliver any value. Instead, leading companies are setting a bold ambition and getting started quickly, but thoughtfully.
The familiar playbook of iterative product development applies here. Applications won’t take off unless they solve a specific customer or employee problem. Once developed, companies would be wise to bring applications to the front line and an initial set of customers in a controlled way. The feedback can then be used to continuously adjust the product until it’s ready to be scaled. We’re talking weeks, not months—and definitely not years. As the first application scales up, the company can already be developing additional applications that solve customers’ next two problems. What’s different from “traditional” digital products is that early generative AI use cases will require continuous improvement as new modalities unlock ways to improve or expand applications.
The result is a series of new frontline implementations that in short order start to deliver actual value to customers and real competitive advantage, on top of the organization learning how to develop these changes. This will assure that all efforts have purpose and work toward the audacious goal of changing the standards of their industry, while leaving room for some efforts to fail fast, their lessons informing future generative AI development.
3. Create guardrails and management processes to do this responsibly
Every telco is faced with the same imperative to ensure that adopting AI doesn’t compromise the organization’s data and intellectual property security, customer data security, brand credibility, and legal protections. Early generative AI adopters at the enterprise level are creating their approach to responsible AI in parallel with building out use cases and MVPs. And this could of course change as regulators and governments examine standards globally. For an early innovator, this requires collaboration between leaders from operations, the technology and data team, and the legal department to create the right guardrails that empower—and don’t hamper—the organization. At the same time, early users have found it’s critical to design processes that allow the working team to quickly escalate and resolve issues as they occur.
As Vodafone enabled its data, technology, and marketing teams to adopt nongenerative machine learning and automation tools, company leaders realized they needed to train their teams and improve the organization’s capabilities in order to use AI responsibly. With impending regulations across the globe, Vodafone needed an approach that could work across its operating companies while supporting centrally developed capabilities, and these approaches had to be auditable and meet compliance requirements. The company developed an operating model that allowed it to assess the level of scrutiny required for different types of AI applications and use cases, and it created a framework for the roles and responsibilities of various participants and stakeholders (including data scientists; technology developers; vendors; legal, compliance, and audit teams; investor relations; and human resources). This gave Vodafone leaders the confidence to pursue AI initiatives knowing that they had the right scaffolding in place to scale up their efforts responsibly.
Stepping back, the opportunity at hand with generative AI is both incredibly complex and simple. Telcos, like any organization, have a lot of work ahead to figure out the right governance, guardrails, prototype delivery system, operating rhythms, change management muscles, and prioritization of use cases. But at the same time, the stakes are clear: There’s a limited window during which the telecommunications sector as a whole has a chance to innovate faster than other industries and for individual telcos to move faster than competitors to execute a bold vision. Regardless of the details of each company's approach, the most effective leaders will channel their organization’s energy toward what’s doable with AI today and identifying how they can wield it to systematically and persistently improve their business.
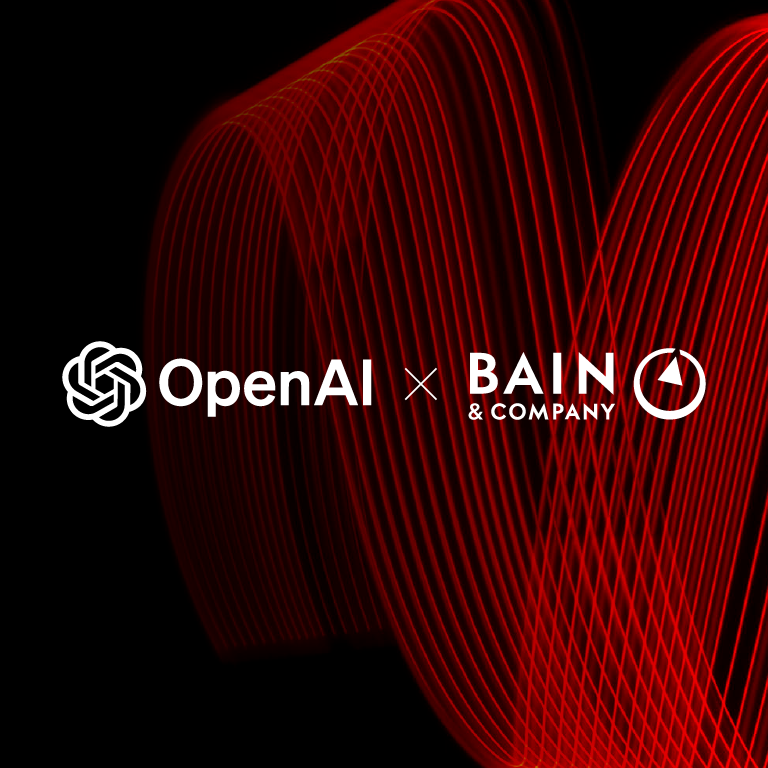
Better Together: OpenAI and Bain Form an Alliance
Harness the power of generative AI to transform your business