Report
This article is part of Bain's 2021 Technology Report.
The computing industry has gone through three major transitions over the last half-century: the mainframe era; the shift to PC-server computing; and the rise of cloud, mobile, and the Internet. Now, we’re on the cusp of a fourth transition, data-centric computing, which will be powered largely by the increasing pervasiveness of artificial intelligence.
Spurred by a need to process a dramatic growth of unstructured data and enabled by a drastic reduction in the cost of computing, artificial intelligence (AI) tools are becoming more widely available through cloud platforms and open-source software. It’s not hyperbole to say that machines will be able to program machines in the coming years. That will unleash unprecedented efficiency gains for data centers and allow for much broader use of AI technology. Questions about job loss and ethics will remain a feature of the landscape for AI companies, yet it’s also increasingly clear AI will continue to improve products and customer experiences through personalization, create new categories of products and jobs, and enable advances in critical areas of society, such as cybersecurity and public safety.
Now, the real question is, who will lead the way in AI?
The reality check
The common narrative is that AI’s trajectory over the next several years will be shaped by enterprises in all industries rapidly adopting the technology. In reality, it's clear that large cloud service providers (CSPs) will continue to fuel the industry as both its leading adopters and innovators, helping other enterprises customize and deploy their own AI-powered products and services.
Few companies are qualified to be leaders in raw AI innovation. The CSPs are best positioned because of the significant head start they have in using AI on a large scale. This is true across applications such as natural language processing (think Amazon Alexa), image recognition and processing (Facebook, Google), recommendation systems (Google search, Alibaba e-commerce), vision processing in autonomous driving (Alphabet’s Waymo), and smart cities (Alibaba City Brain, Tencent WeCity).
CSPs’ cloud and digital services have given them access to the enormous amounts of data required to effectively train AI models. The exponential growth in the size and complexity of AI models requires AI practitioners that have the ability to build and operate bespoke, large-scale systems. And the CSP business model benefits from the personalization of services that AI can deliver.
CSPs have the largest fleets of AI servers, and their spending on AI computing architecture is accelerating. More than 15% of leading CSPs’ servers are currently focused on AI computing workloads, and that’s expected to rise to more than 30% by 2025, according to Bain interviews with the companies. By then, the top-four AI workloads—vision, natural language processing, recommendation engines, and intelligent search—will account for around 70% of CSPs’ AI server fleets (see Figure 1).
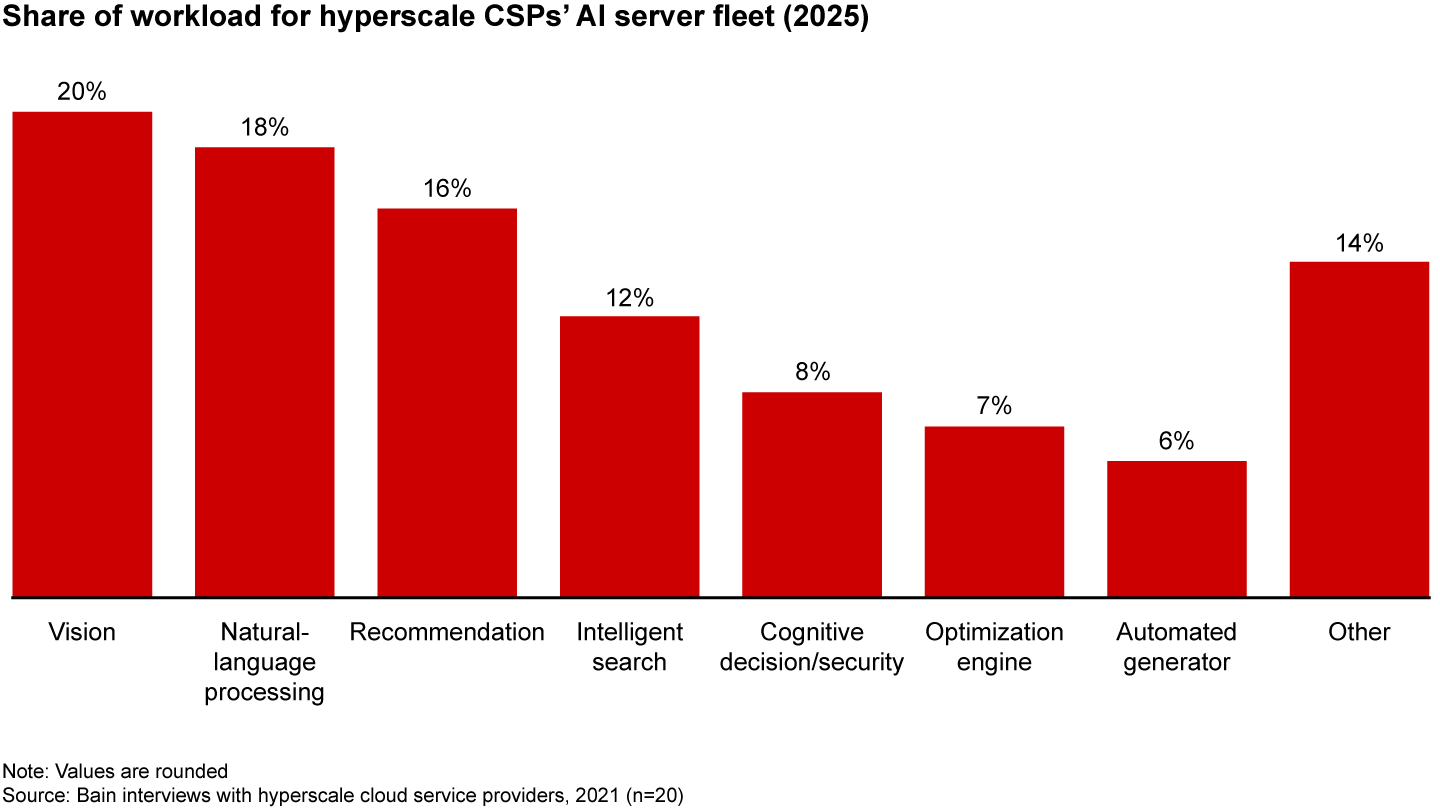
CSPs have also developed some of the most sophisticated commercial AI models. The complexity of deep-learning models is more than doubling every three to four months (see Figure 2). Among the largest ones are Google’s 1.6-trillion parameter Switch Transformer model introduced in January, and OpenAI’s 175 billion-parameter GPT-3 model introduced in May 2020 and commercialized by Microsoft in May of this year.
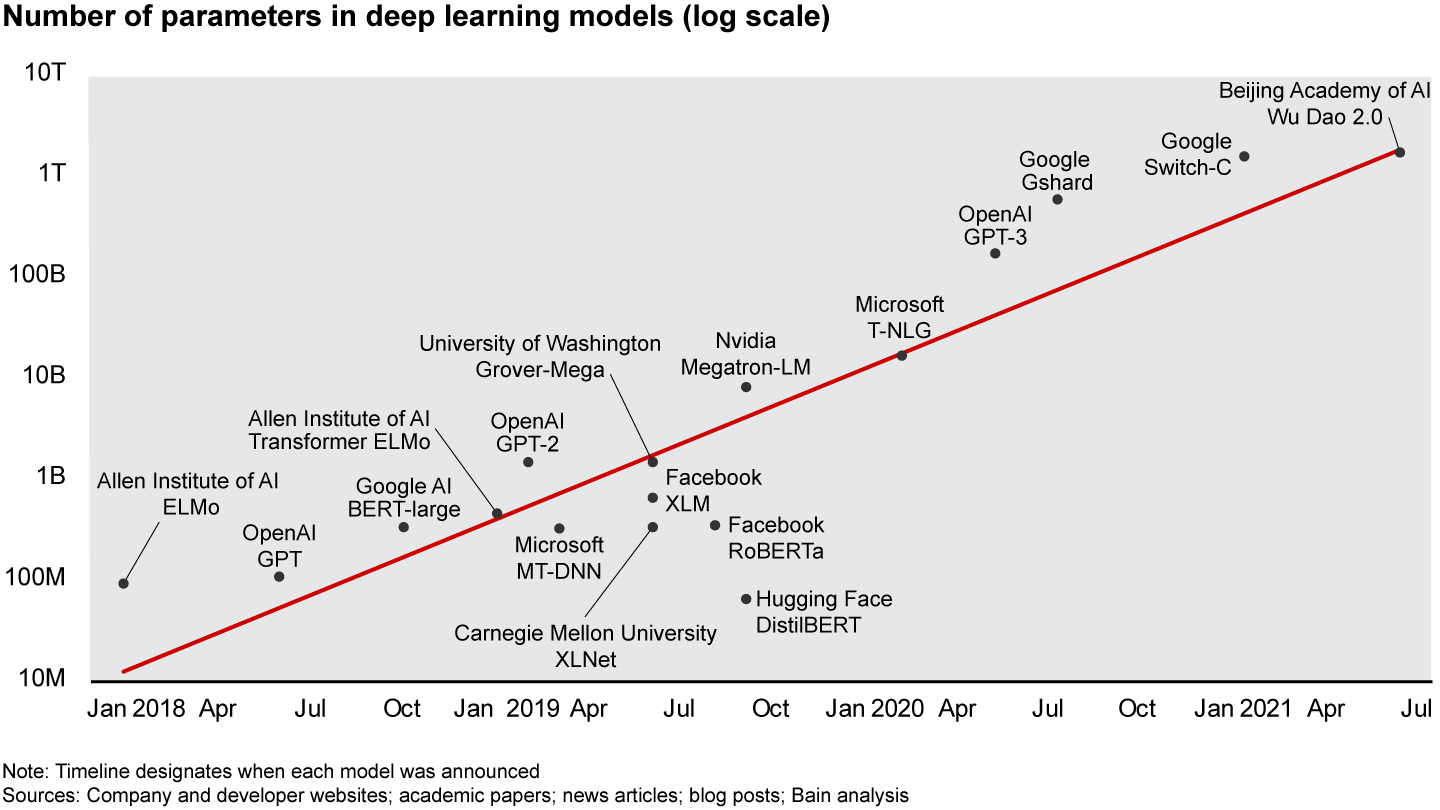
CSPs created and advanced the leading AI developer frameworks, which are collections of math libraries packaged to support developer training and inference of complex AI neural net models. The vast majority of AI algorithms run on TensorFlow (Google), PyTorch (Facebook), and PaddlePaddle (Baidu), and millions of AI developers worldwide rely heavily on this foundation laid by the CSPs.
Furthermore, CSPs are among the most prolific developers of AI-enabled services. Amazon Web Services, Microsoft Azure, Google Cloud Platform, and Alibaba Cloud expanded their AI platform offerings by about 30% from September 2020 to June 2021 (see Figure 3). Notable advances are being made not only in core AI models and services, but also more sophisticated text-to-speech capabilities and image analysis. CSPs are also introducing tools to simplify the full AI workflow, from data ingestion through model deployment and maintenance. For example, AWS has expanded its SageMaker services to simplify each stage of the AI development life cycle.
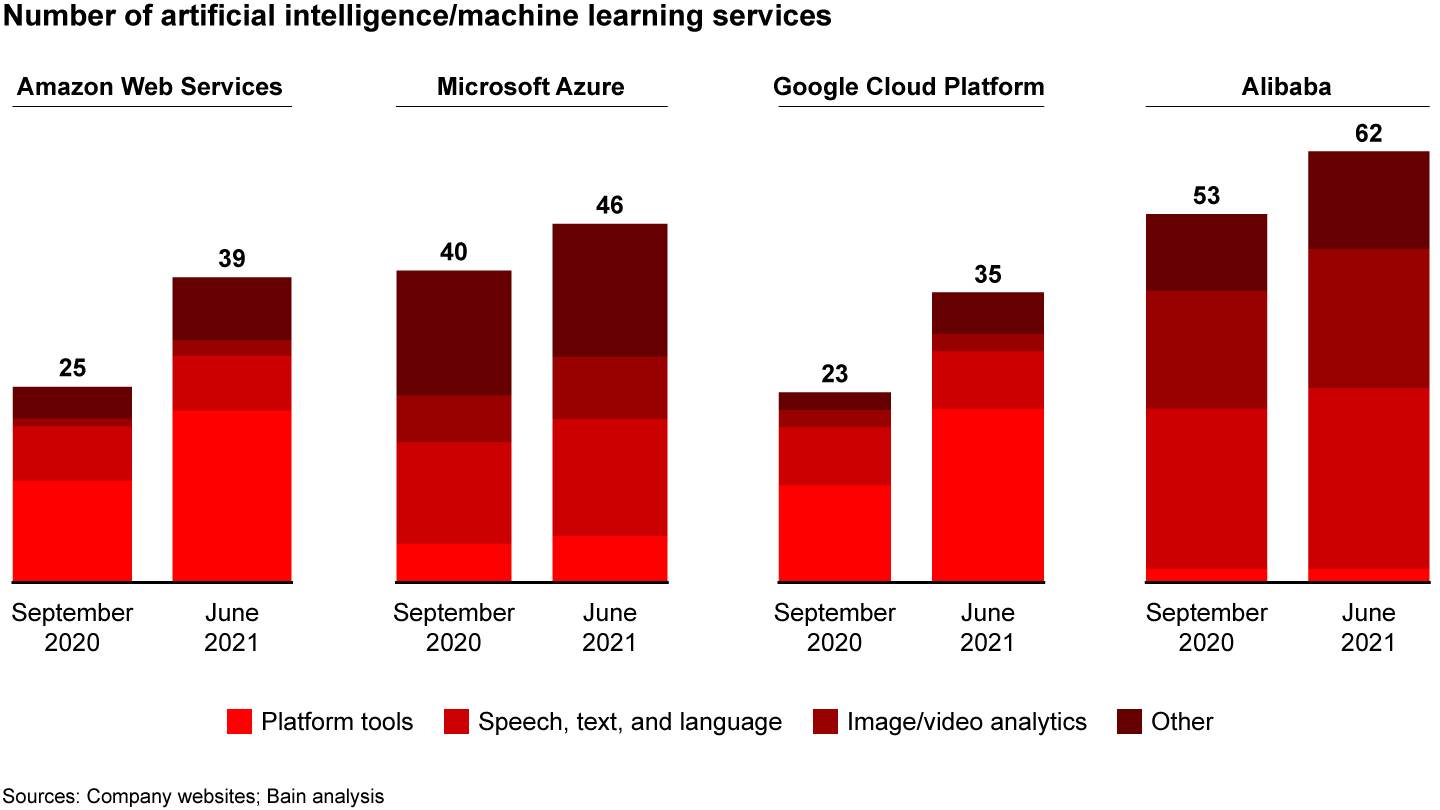
Lastly, much of the AI talent is concentrated within CSPs. The number of AI employees at the top five US hubs (Amazon, Microsoft, Alphabet, Facebook, and IBM) exceeds the size of the AI workforces at the next 45 US companies combined.
All of this points to CSPs likely remaining the primary vehicle through which AI capabilities are turned into products and made widely available. As this democratization of AI accelerates, fewer companies will build their own AI stacks because the time and money required often won’t be worth it. Some still will do so for business cases where CSPs’ AI tools lack particular features or domain-specific functionality, proprietary and differentiating data sets exist, or when there are privacy and security considerations. But this will increasingly be the exception, not the rule.
Enterprises will be able to build differentiating AI products and services using third-party tools—usually from the CSPs—allowing them to focus on adding value through domain-specific expertise. For example, Intuit engineers are using AWS SageMaker’s AI model design and training tools to help incorporate AI into the company’s financial software products. This has cut Intuit’s typical AI development timeline from six months to less than a week, and in 2020 the company increased its number of AI models in production by 50%, to several hundred. Most importantly, it has helped Intuit save customers time through better self-help software tools and receipt processing, and it has enabled the company to underwrite more loans by improving repayment forecasting.
Choosing a path
So, what does all of this mean for technology providers?
For CSPs, the top priority is staying ahead by continuing to build data sets and maximizing the value of AI in the core business. The race is on to win enterprise AI computing workloads and data sets, which can be fed into the cloud platform to generate new insights that, in turn, help improve AI products.
But CSPs should choose wisely when going after new industries and product adjacencies, balancing near-term return on investment with long-term product differentiation. Success in different industries often requires significant domain expertise and high technical performance. In many cases, forming partnerships with system integrators and third parties will go a long way.
Leading CSPs also recognize it’s important to continue putting in the hard work of building trustworthy relationships and data policies, guiding public understanding and regulations of AI, and developing advanced technology to reduce bias and preserve privacy in AI products. The leaders understand these efforts can pay off not only for the companies themselves, but the AI field as a whole.
Meanwhile, even though technology companies in disrupted industries might not be leaders in raw AI innovation, they can still find innovative ways to apply others’ foundational AI tools and services to better serve their own customers. It helps that these companies have some of the winning characteristics of the AI era. These businesses—which include enterprise software firms, system integrators, and technology companies serving manufacturing, retail, healthcare, and other specific sectors—have many customer touchpoints and/or are frequently working with unstructured data.
The emerging leaders in this segment are thinking several steps ahead. While it can’t hurt to hire data scientists and chief digital officers, that’s no substitute for deeply understanding how AI will transform the value of the company’s products to customers. The leaders will develop a customer-led roadmap that prioritizes their desired AI uses and takes advantage of user feedback loops to accelerate product design cycles, enhance products, and improve customer success.
As for the AI computing enablers—semiconductor developers and manufacturers—it’s clear now that AI will be the defining computing workload that underpins their success after the cloud and mobile era. The explosion of AI workloads and the field’s dynamics for innovation and democratization led many to declare the death of the general-purpose (GP) processor, such as CPUs. But GP processors actually have a bright future, with targeted adaptations that will likely involve a family of processors better suited to handle the requirements of AI and other critical workloads.
Lastly, many technology hardware vendors have already started the journey of evolving their business model, moving from hardware to software to hyperconverged, integrated “delivery-as-a-service.” The proliferation of AI increases the urgency to accelerate this journey.
Companies in this segment are considering turning their traditional hardware products into a vehicle for delivering CSPs’ AI software stacks for converged hardware, which could prove powerful in combination with the tech hardware vendors’ enterprise expertise and customer reach. Many enterprise customers have the data science and application engineering skills required for their industry’s AI uses, but their capabilities aren’t as strong in quality of delivery services, server fleet management, and system-level technology operations. At the same time, CSPs, especially in the US, are mostly staying away from being the full AI delivery vehicle for these enterprises because they don’t want to get dirt under their fingernails handling all the operations-heavy work of installation, retrofitting, system integration, and maintenance. This could be the sweet spot for the hardware tech vendors, but it would require a swifter shift to a business model based heavily on operational services.
Nevertheless, these vendors will still have opportunities to enhance their existing hardware products with AI-enabled remote monitoring and adaptive improvement capabilities, so they can take an even more active role in helping their customers get the most out of their products.
Ultimately, the AI battleground is still being shaped. But everyone has a viable path to success, from the raw innovation leaders to the many companies building products and services on top. Guided by their company’s strengths, the emerging leaders are moving quickly to establish a foothold in this new era.